Cross-modal correlation learning method based on multi-granularity hierarchical network
A learning method and cross-modal technology, applied in neural learning methods, biological neural network models, multimedia data retrieval, etc., can solve the problems of ignoring supplementary effects, ignoring rich fine-grained information, and unable to fully balance the associated learning process, etc. The effect of improving accuracy
- Summary
- Abstract
- Description
- Claims
- Application Information
AI Technical Summary
Problems solved by technology
Method used
Image
Examples
Embodiment Construction
[0022] The present invention will be further described in detail below in conjunction with the accompanying drawings and specific embodiments.
[0023] A cross-modal association learning method based on a multi-granularity hierarchical network of the present invention, its process is as follows figure 1 shown, including the following steps:
[0024] (1) Establish a cross-modal database containing multiple modal types, and divide the database into a training set, a verification set, and a test set, process the data of different modalities in the database, and extract all modal original The data and the eigenvectors of the partitioned data.
[0025] In this embodiment, the cross-modal database may include multiple modality types, and different block processing methods are used for different modality data to divide the original data into multiple parts. Taking images and texts as examples, the Selective Search algorithm is used to extract multiple candidate areas containing ric...
PUM
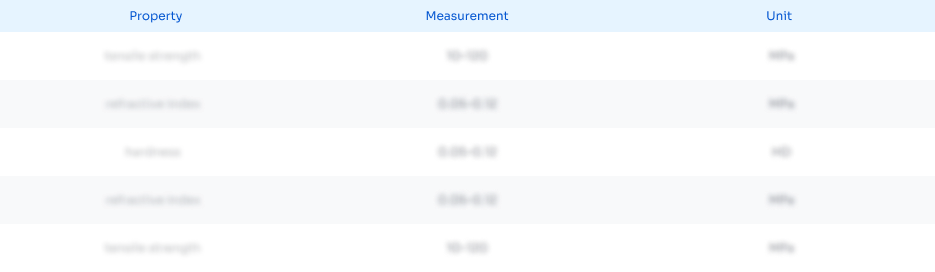
Abstract
Description
Claims
Application Information
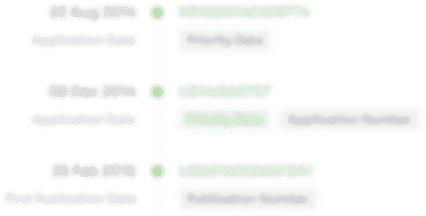
- R&D Engineer
- R&D Manager
- IP Professional
- Industry Leading Data Capabilities
- Powerful AI technology
- Patent DNA Extraction
Browse by: Latest US Patents, China's latest patents, Technical Efficacy Thesaurus, Application Domain, Technology Topic, Popular Technical Reports.
© 2024 PatSnap. All rights reserved.Legal|Privacy policy|Modern Slavery Act Transparency Statement|Sitemap|About US| Contact US: help@patsnap.com