Deep intelligent driving application-oriented traffic sign recognition algorithm
A technology of traffic sign recognition and traffic sign, which is applied in the field of traffic sign recognition algorithm, can solve the problems of timeliness, poor stability, low scalability and versatility, and achieve the effect of improving scalability
- Summary
- Abstract
- Description
- Claims
- Application Information
AI Technical Summary
Problems solved by technology
Method used
Image
Examples
Embodiment Construction
[0030] Such as figure 1 As shown, a traffic sign recognition algorithm for deep smart driving applications, specifically includes the following steps:
[0031]1), firstly perform data enhancement on the multi-source public beta dataset;
[0032] 2), and then alternately iteratively train the detection network and the recognition network;
[0033] 3) Using the detection data set GTSDB and LISA-TS as the data platform, based on the multi-layer convolution feature of the image, use the top-down multi-scale convolution feature fusion method to construct a set of layers representing the convolution of different scales of the image Semantic feature maps to enable anchor points to extract regions of interest for traffic signs on multi-scale convolutional feature maps with more semantic sets;
[0034] 4) Design and study the series and parallel collection methods of the middle layer of the network. Through comparative experiments, dig out and reveal the internal learning mechanism o...
PUM
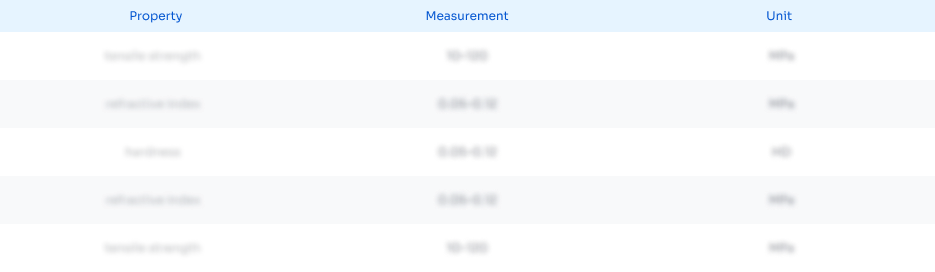
Abstract
Description
Claims
Application Information
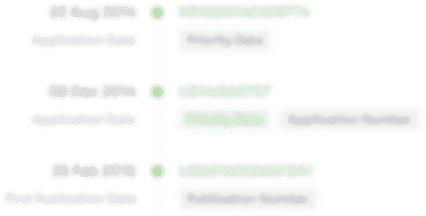
- R&D Engineer
- R&D Manager
- IP Professional
- Industry Leading Data Capabilities
- Powerful AI technology
- Patent DNA Extraction
Browse by: Latest US Patents, China's latest patents, Technical Efficacy Thesaurus, Application Domain, Technology Topic, Popular Technical Reports.
© 2024 PatSnap. All rights reserved.Legal|Privacy policy|Modern Slavery Act Transparency Statement|Sitemap|About US| Contact US: help@patsnap.com