Multi-spectral image classification method based on threshold self-adaption and convolutional neural network
A convolutional neural network and multi-spectral image technology, applied in the field of image processing, can solve the problems of difficulty in obtaining high classification accuracy of multi-spectral images, low spectral resolution of multi-spectral data bands, and difficulty in classifying complex types of ground objects, etc. To achieve the effect of improving classification accuracy, redundant information, and large amount of data
Active Publication Date: 2017-11-24
XIDIAN UNIV
View PDF7 Cites 24 Cited by
- Summary
- Abstract
- Description
- Claims
- Application Information
AI Technical Summary
Problems solved by technology
[0006] However, none of the above classification methods take into account that the multispectral data has fewer bands and low spectral resolution. Not only is the data volume large, but it is also difficult to classify complex types of ground objects. Therefore, it is difficult to obtain high classification accuracy
Method used
the structure of the environmentally friendly knitted fabric provided by the present invention; figure 2 Flow chart of the yarn wrapping machine for environmentally friendly knitted fabrics and storage devices; image 3 Is the parameter map of the yarn covering machine
View moreImage
Smart Image Click on the blue labels to locate them in the text.
Smart ImageViewing Examples
Examples
Experimental program
Comparison scheme
Effect test
Embodiment
[0091] Simulation conditions:
[0092] The hardware platform is: HPZ840.
[0093] The software platform is: MX-Net.
the structure of the environmentally friendly knitted fabric provided by the present invention; figure 2 Flow chart of the yarn wrapping machine for environmentally friendly knitted fabrics and storage devices; image 3 Is the parameter map of the yarn covering machine
Login to View More PUM
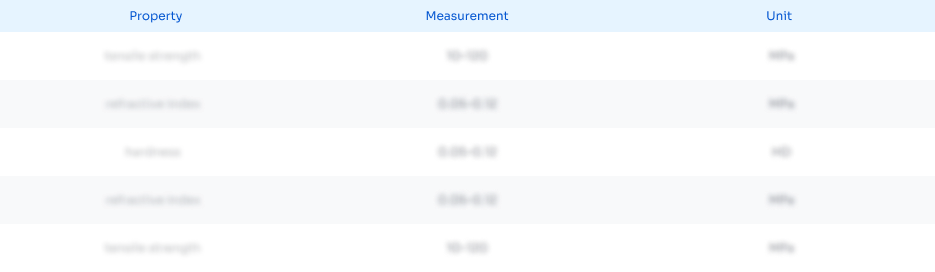
Abstract
The invention discloses a multi-spectral image classification method based on threshold self-adaption and a convolutional neural network. The method comprises the following steps of inputting multispectral images of different time phases and different wave bands of satellites to be classified, and carrying out normalization on all pixels of a marked portion of a same wave band image in all cities; stacking selected 9 wave bands into one image and taking the image as a training data set; constructing a classification model based on the convolutional neural network, using the training data set to train the classification model so as to acquire a probability model based on OSM, using the model and a confidence coefficient strategy to adjust a softmax output result and acquiring a final classification model, and finally, uploading a test result to an IEEE website so as to acquire classification accuracy. By using the multi-spectral image classification method, characteristics that there are a lot of wave bands, a data volume is large and there are a lot of information redundancy in the multispectral images are fully used so that a problem that surface features of complex types are difficult to classify is solved, classification accuracy is increased, an error dividing rate is reduced and a classification speed can be increased.
Description
technical field [0001] The invention belongs to the technical field of image processing, and in particular relates to a multi-source, multi-temporal, multi-mode multispectral image classification method based on threshold self-adaptation and convolutional neural network. Background technique [0002] Multispectral images refer to the images formed by the reflection and transmission of electromagnetic waves of any band by objects, including visible light, infrared rays, ultraviolet rays, millimeter waves, X-rays, and gamma-ray reflection or transmission images. Multispectral image fusion refers to combining the multispectral image information features of the same scene obtained from multispectral detectors, and using their temporal and spatial correlation and information complementarity to obtain a more comprehensive and clear description of the scene. . For example, infrared images and visible light images are complementary: to the human eye, visible light has rich details ...
Claims
the structure of the environmentally friendly knitted fabric provided by the present invention; figure 2 Flow chart of the yarn wrapping machine for environmentally friendly knitted fabrics and storage devices; image 3 Is the parameter map of the yarn covering machine
Login to View More Application Information
Patent Timeline
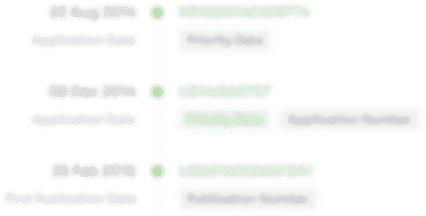
IPC IPC(8): G06K9/00G06K9/62G06N3/04
CPCG06V20/194G06V20/13G06N3/045G06F18/213G06F18/2415
Inventor 焦李成屈嵘孙莹莹唐旭杨淑媛侯彪马文萍刘芳尚荣华张向荣张丹马晶晶
Owner XIDIAN UNIV
Who we serve
- R&D Engineer
- R&D Manager
- IP Professional
Why Patsnap Eureka
- Industry Leading Data Capabilities
- Powerful AI technology
- Patent DNA Extraction
Social media
Patsnap Eureka Blog
Learn More Browse by: Latest US Patents, China's latest patents, Technical Efficacy Thesaurus, Application Domain, Technology Topic, Popular Technical Reports.
© 2024 PatSnap. All rights reserved.Legal|Privacy policy|Modern Slavery Act Transparency Statement|Sitemap|About US| Contact US: help@patsnap.com