A Real-time Detection Method of Pedestrians and Vehicles Based on Lightweight Deep Network
A deep network, real-time detection technology, applied in the field of deep learning technology, can solve the problems of low accuracy, large number of parameters, too large number of parameters, etc., to achieve good practicability and real-time performance, small number of model parameters, accurate detection high degree of effect
- Summary
- Abstract
- Description
- Claims
- Application Information
AI Technical Summary
Problems solved by technology
Method used
Image
Examples
Embodiment Construction
[0030] The present invention proposes a new convolutional neural network (CNN) structure to complete the feature extraction part of pedestrians and vehicles. The specific network structure is as figure 1 Shown:
[0031] The network consists of 8 layers in total (5 meta-modules + 3 convolutions). Different from the linear structure of VGG-16, this network adopts a skip connection method between layers to fuse the shallower features of the network with deeper features: the first convolutional layer outputs the feature spectrum to the first meta-module , the convolutional layer extracts features from the image through a series of filters, and adjusts the parameters through regularization and activation operations to make the network converge better. The first meta-module outputs the feature spectrum to the second meta-module, and the first convolutional layer The output feature spectrum is fused with the output feature spectrum of the second meta-module and then output to the t...
PUM
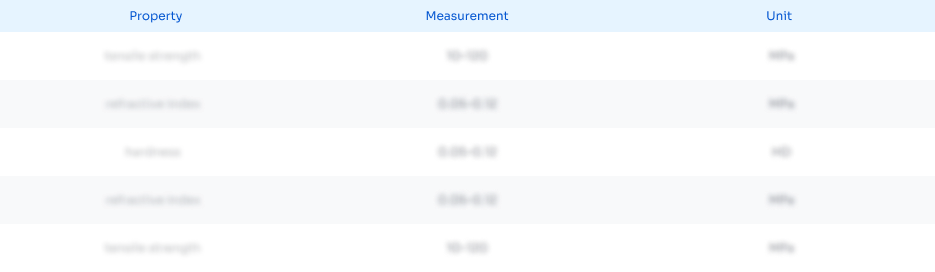
Abstract
Description
Claims
Application Information
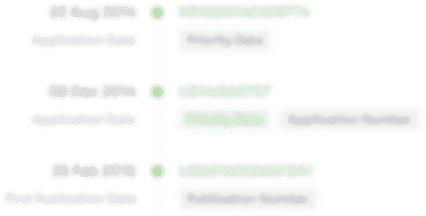
- R&D Engineer
- R&D Manager
- IP Professional
- Industry Leading Data Capabilities
- Powerful AI technology
- Patent DNA Extraction
Browse by: Latest US Patents, China's latest patents, Technical Efficacy Thesaurus, Application Domain, Technology Topic, Popular Technical Reports.
© 2024 PatSnap. All rights reserved.Legal|Privacy policy|Modern Slavery Act Transparency Statement|Sitemap|About US| Contact US: help@patsnap.com