Building load forecasting method and device based on improved IHCMAC neural network
A load forecasting and neural network technology, applied in neural learning methods, biological neural network models, geometric CAD, etc., can solve problems such as unsatisfactory forecasting effect and affecting forecasting effect, and achieve the improvement of global search ability, strong generalization ability, Universal effect
- Summary
- Abstract
- Description
- Claims
- Application Information
AI Technical Summary
Problems solved by technology
Method used
Image
Examples
Embodiment 1
[0071] This embodiment discloses a building load forecasting method based on an improved IHCMAC neural network, including:
[0072] Step 1: Simulate the actual operation of the building to obtain building cooling / heating load data and its influencing factors;
[0073] Simulation data include building cooling / heating load data and influencing factors of building cooling / heating load, said influencing factors include environmental parameters (outdoor temperature, outdoor humidity, outdoor wind speed, solar radiation intensity) and personnel distribution data (occupancy rate) .
[0074] In this example, an office building located in Jinan City is used as a building prototype in this paper, and the building model is constructed by using SketchUp software, and the actual operation of the building is simulated with the help of TRNSYS simulation operation software, and the energy consumption characteristics of the building are analyzed. The relevant parameters of the building are as ...
Embodiment 2
[0105] The purpose of this embodiment is to provide a computing device.
[0106] Based on the above purpose, the present embodiment provides a building load forecasting device based on the improved IHCMAC neural network, including a memory, a processor, and a computer program stored in the memory and operable on the processor, and the processor executes the Perform the following steps when describing the program:
[0107] Receive the building cooling / heating load data and its influencing factors data obtained by simulating the actual operation of the building;
[0108] Determine the input variables of the model according to the degree of correlation between the influencing factors and the building cooling / heating load;
[0109] According to the particle swarm-K-means clustering algorithm, the input variables are clustered, and the values of the L cluster centers are obtained, which are the model node values, and a Gaussian kernel function is defined for each node;
[0110]...
Embodiment 3
[0112] The purpose of this embodiment is to provide a computer-readable storage medium.
[0113] Based on the above purpose, this embodiment provides a computer-readable storage medium, on which a computer program is stored, and when the program is executed by a processor, the following steps are performed:
[0114] Receive the building cooling / heating load data and its influencing factors data obtained by simulating the actual operation of the building;
[0115] Determine the input variables of the model according to the degree of correlation between the influencing factors and the building cooling / heating load;
[0116] According to the particle swarm-K-means clustering algorithm, the input variables are clustered, and the values of the L cluster centers are obtained, which are the model node values, and a Gaussian kernel function is defined for each node;
[0117] The weight of the nodes is updated through the weight training algorithm to obtain the building load predict...
PUM
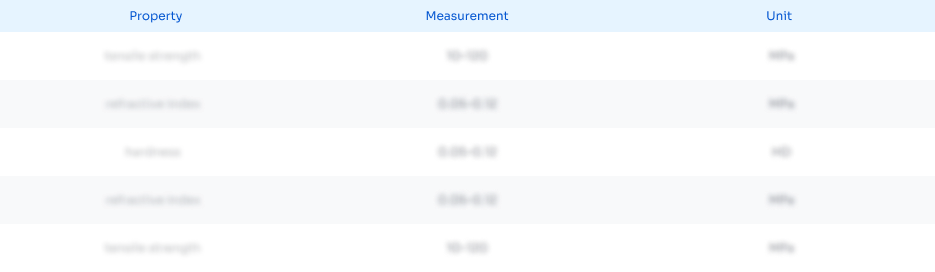
Abstract
Description
Claims
Application Information
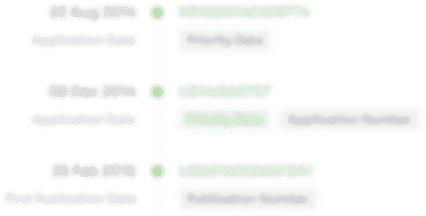
- R&D Engineer
- R&D Manager
- IP Professional
- Industry Leading Data Capabilities
- Powerful AI technology
- Patent DNA Extraction
Browse by: Latest US Patents, China's latest patents, Technical Efficacy Thesaurus, Application Domain, Technology Topic.
© 2024 PatSnap. All rights reserved.Legal|Privacy policy|Modern Slavery Act Transparency Statement|Sitemap