Vehicle type identification method and system based on deep neural network
A deep neural network, vehicle recognition technology, applied in biological neural network models, neural learning methods, character and pattern recognition, etc.
- Summary
- Abstract
- Description
- Claims
- Application Information
AI Technical Summary
Problems solved by technology
Method used
Image
Examples
Embodiment
[0146] The vehicle type recognition method of the present embodiment is divided into two steps: the first step is to carry out model training on various types of vehicles; The network is synchronized.
[0147] The first step: model training phase
[0148] 1] Build a vehicle sample set
[0149] There are a total of 2,234 vehicle brands and more than 2,000 types of vehicle styles collected, and about 1,000 to 2,000 samples of each type of vehicle, totaling about 5 million. All samples are obtained through bayonet capture, and each sample contains a clear and complete color photo of a vehicle. Considering that too low resolution will increase the error rate of vehicle identification, the resolution of each vehicle photo should be at least It is 2048*1536, and the size is at least 3 million pixels.
[0150] 2] Sample pretreatment
[0151] 2.1] Sample expansion
[0152] 2.1.1] Scale the size of all sample images to 512*384 pixels (the vehicle photos are zoomed without cropping...
PUM
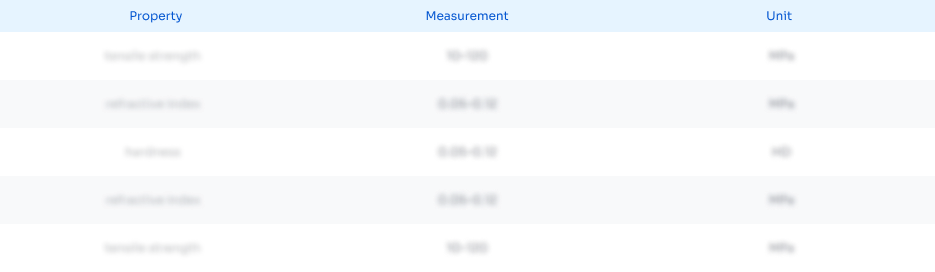
Abstract
Description
Claims
Application Information
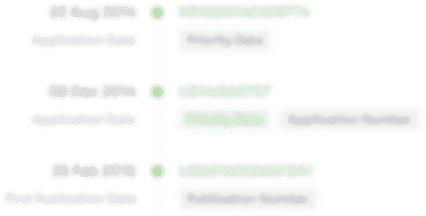
- R&D Engineer
- R&D Manager
- IP Professional
- Industry Leading Data Capabilities
- Powerful AI technology
- Patent DNA Extraction
Browse by: Latest US Patents, China's latest patents, Technical Efficacy Thesaurus, Application Domain, Technology Topic, Popular Technical Reports.
© 2024 PatSnap. All rights reserved.Legal|Privacy policy|Modern Slavery Act Transparency Statement|Sitemap|About US| Contact US: help@patsnap.com