Maize leaf disease identification method based on convolutional neural network model GoogleNet
A convolutional neural network and disease identification technology, applied in the field of image recognition, can solve the problems of low identification accuracy of disease types and many network model parameters, and achieve the effect of improving identification accuracy and loss
- Summary
- Abstract
- Description
- Claims
- Application Information
AI Technical Summary
Problems solved by technology
Method used
Image
Examples
specific Embodiment approach 1
[0017] Specific implementation mode one: as figure 1 As shown, the corn leaf disease identification method based on the convolutional neural network model GoogleNet includes the following steps:
[0018] Step 1: Collect the corn leaf image data set, expand the collected corn leaf image data set, and then preprocess the image data set; the preprocessed image data set is divided into a training set and a test set;
[0019] Step 2: Input the training set and test set preprocessed in step 1 into the convolutional neural network model GoogleNet, use the first classifier for training and testing, adjust the parameters of the convolutional neural network model GoogleNet, and obtain an optimized convolutional neural network Network model GoogleNet (optimal top-1 accuracy and minimum model loss);
[0020] Step 3: Input the test set processed in step 1 into the optimized convolutional neural network model GoogleNet obtained in step 2 to complete the identification of corn leaf diseases...
specific Embodiment approach 2
[0022] Embodiment 2: The difference between this embodiment and Embodiment 1 is that the specific process of expanding the collected corn leaf image data set in the step 1 is as follows:
[0023] Rotate the images in the corn leaf image dataset by 90°, 180°, and 270° (each image is rotated by 90°, 180°, and 270°, and one image becomes four images after rotation), and the The rotated image is mirrored, and the center of the mirrored image is divided according to the same size; the rotated, mirrored and segmented images are converted into grayscale images in turn (the corn leaf image data set processed by the above steps Part of the image is converted to grayscale).
[0024] Other steps and parameters are the same as those in Embodiment 1.
specific Embodiment approach 3
[0025] Embodiment 3: The difference between this embodiment and Embodiment 1 or 2 is that: the specific process of preprocessing the image data set in the step 1 is:
[0026] Use the Python language to normalize the image name, format and size in the input data set based on OpenCv, and mark the normalized image categories. The image categories include 8 leaf disease categories and 1 healthy leaf category. , 8 leaf disease categories are large spot, small spot, rust, brown spot, round spot, Curvularia leaf spot, gray spot and dwarf mosaic.
[0027] Other steps and parameters are the same as those in Embodiment 1 or Embodiment 2.
PUM
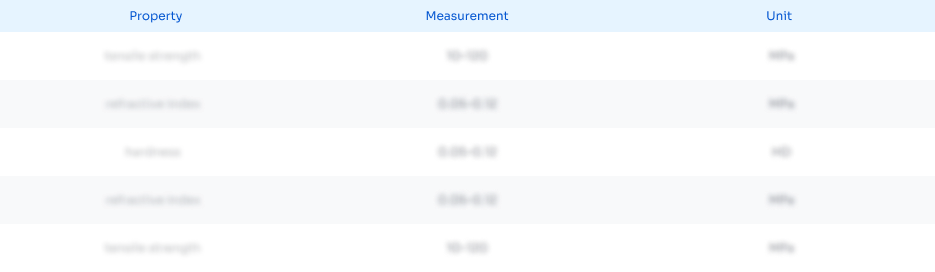
Abstract
Description
Claims
Application Information
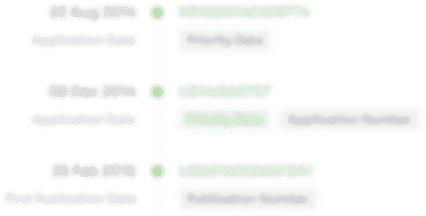
- R&D Engineer
- R&D Manager
- IP Professional
- Industry Leading Data Capabilities
- Powerful AI technology
- Patent DNA Extraction
Browse by: Latest US Patents, China's latest patents, Technical Efficacy Thesaurus, Application Domain, Technology Topic.
© 2024 PatSnap. All rights reserved.Legal|Privacy policy|Modern Slavery Act Transparency Statement|Sitemap