Unstructured data default probability prediction method based on deep learning
An unstructured data and deep learning technology, applied in the field of financial risk control, can solve problems such as difficulty in adapting to the rapid evolution of the online risk environment, difficulty in risk modeling, and complex data transformation, so as to improve financial risk control capabilities and reduce credit effect of risk
- Summary
- Abstract
- Description
- Claims
- Application Information
AI Technical Summary
Problems solved by technology
Method used
Image
Examples
Embodiment Construction
[0043] In order to make the object, technical solution and advantages of the present invention clearer, the present invention is described below through specific embodiments shown in the accompanying drawings. It should be understood, however, that these descriptions are exemplary only and are not intended to limit the scope of the present invention. Also, in the following description, descriptions of well-known structures and techniques are omitted to avoid unnecessarily obscuring the concept of the present invention.
[0044] combine figure 1 Describe this embodiment, the default probability prediction method based on deep learning of unstructured data of the present invention, by mining unstructured data such as text and time series that are not fully utilized in traditional financial risk control, and based on deep learning and big data technology to capture credit Based on the potential risk behavior pattern of the subject, high-dimensional data credit risk modeling is c...
PUM
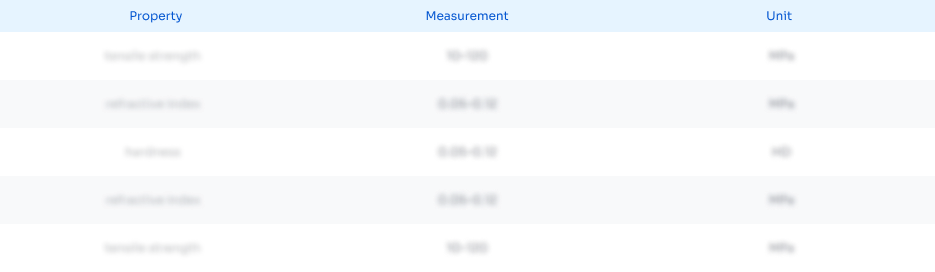
Abstract
Description
Claims
Application Information
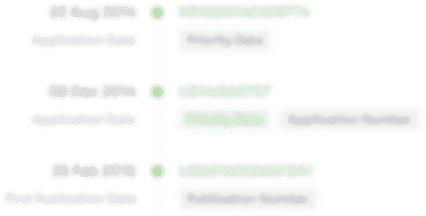
- R&D Engineer
- R&D Manager
- IP Professional
- Industry Leading Data Capabilities
- Powerful AI technology
- Patent DNA Extraction
Browse by: Latest US Patents, China's latest patents, Technical Efficacy Thesaurus, Application Domain, Technology Topic.
© 2024 PatSnap. All rights reserved.Legal|Privacy policy|Modern Slavery Act Transparency Statement|Sitemap