Method for classifying multi-variable time sequences based on deep long/short-term memory neural network
A long-short-term memory and neural network technology, applied in the field of multivariate time series classification based on deep long-term and short-term memory neural network, can solve the problems of long sample time, low classification accuracy, large number of sample features, etc. High adaptability and high precision
- Summary
- Abstract
- Description
- Claims
- Application Information
AI Technical Summary
Problems solved by technology
Method used
Image
Examples
Embodiment 1
[0032] as attached figure 1 as shown, figure 1 is a schematic diagram of the structure of the deep LSTM classification model proposed by the present invention, figure 1 A 3-layer LSTM is used as an example to show the structure of the model.
[0033] A deep recurrent neural network classification model suitable for classification tasks based on long-term short-term memory neurons, using LSTM as the hidden layer of the recurrent neural network, and forming a huge hidden layer state space by stacking LSTM layers, and combining the last LSTM layer The output results are input into the Softmax classifier for classification.
[0034] The long-short-term memory neuron-based recurrent neural network classification model uses a long-short-term memory neuron module including an input gate, an output gate, a forget gate and a state unit, and does not include peephole connections. The long-short-term memory recurrent neural network used, the calculation formula of the output result is...
Embodiment 2
[0053] In this embodiment, for multivariate time series classification problems, a deep recursive neural network model is constructed by using the hidden layer results of a recurrent neural network such as LSTM, and finally a Softmax classifier is used to process the output results of the recurrent neural network, then the multivariable Classify time series data.
[0054] The present invention has universal applicability to time series data sets in various fields such as medicine, machinery, handwriting recognition, language recognition, etc. Now take the speech recognition data set Australian language as an example, which is derived from the UCI machine learning database [K.Bache and M.Lichman, "UCI machine learning repository," 2013. https: / / archive.ics.uci.edu / ml / datasets.html.], the Australian language dataset has a total of 2565 samples, and the number of variables in each sample is 22, the sample length is between [45,136], and belongs to one of the 95 categories. In or...
PUM
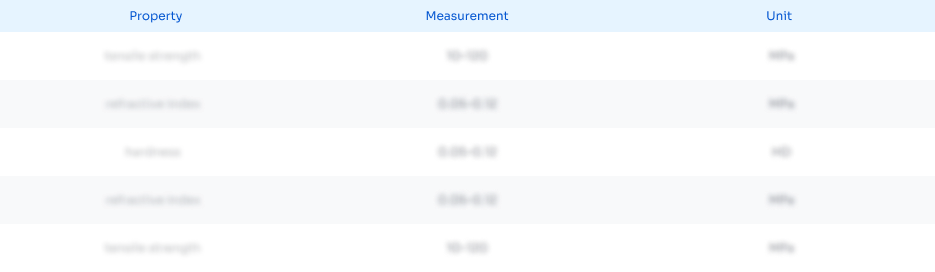
Abstract
Description
Claims
Application Information
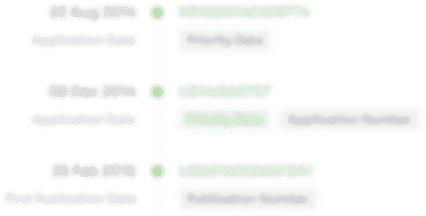
- R&D Engineer
- R&D Manager
- IP Professional
- Industry Leading Data Capabilities
- Powerful AI technology
- Patent DNA Extraction
Browse by: Latest US Patents, China's latest patents, Technical Efficacy Thesaurus, Application Domain, Technology Topic.
© 2024 PatSnap. All rights reserved.Legal|Privacy policy|Modern Slavery Act Transparency Statement|Sitemap