Higher level brain glioma survival period prediction method based on sparse representation framework and higher level brain glioma survival period prediction system based on sparse representation framework
A sparse representation, glioma technology, applied in the field of medical image processing, can solve problems such as limiting the comprehensive utilization of deep pathological features
- Summary
- Abstract
- Description
- Claims
- Application Information
AI Technical Summary
Problems solved by technology
Method used
Image
Examples
Embodiment Construction
[0034] The following are the specific implementation steps of the whole method:
[0035] 1. Firstly, perform operations such as brain removal and gray-scale normalization on the images in the data set, and select 30 images from the T1-enhanced and T2-weighted MRI image collections for manual labeling of tumor areas, and then send the labeling results and corresponding images to Two kinds of convolutional neural networks constructed were used to train the network parameters, and finally the two trained convolutional neural networks were used to segment the tumor area of the corresponding modality image.
[0036] 2. Extract the local SIFT features of the two modalities of each patient, and the key points of the SIFT features are evenly distributed in each layer of the tumor image at intervals of 8 pixels. For each key point, first divide the 16*16 pixel neighborhood into 16 4*4 pixel sub-neighborhoods, then calculate the gradient size and direction of each sub-neighborhood, an...
PUM
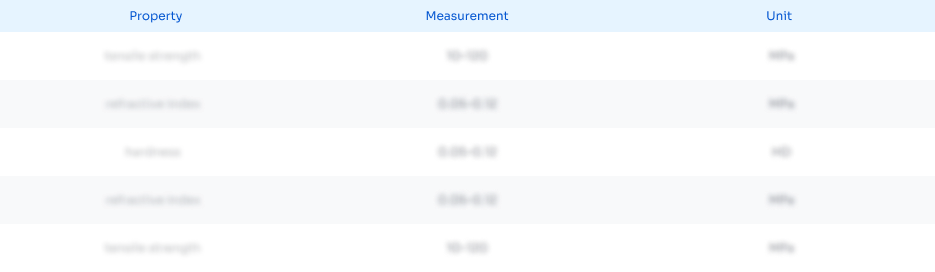
Abstract
Description
Claims
Application Information
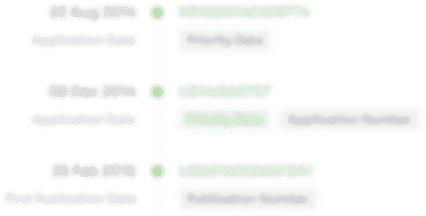
- R&D Engineer
- R&D Manager
- IP Professional
- Industry Leading Data Capabilities
- Powerful AI technology
- Patent DNA Extraction
Browse by: Latest US Patents, China's latest patents, Technical Efficacy Thesaurus, Application Domain, Technology Topic.
© 2024 PatSnap. All rights reserved.Legal|Privacy policy|Modern Slavery Act Transparency Statement|Sitemap