Automatic segmentation method for pre-background of lepidopteron image based on full convolutional neural network
A convolutional neural network, lepidopteran technology, applied in image analysis, image enhancement, image data processing, etc., to improve accuracy and efficiency, reduce labor costs, and eliminate background interference
- Summary
- Abstract
- Description
- Claims
- Application Information
AI Technical Summary
Problems solved by technology
Method used
Image
Examples
example 1
[0057] 1. Use the matting function module attached to "Light and Shadow Magic Hand" or the GrabCut+Lazy Snapping tool to interactively remove the background of the training and test sets of insect specimen images, and set the background to black and the foreground to white to obtain the front The target image for background segmentation.
[0058] 2. Randomly select 80% of the data set as training data, and the remaining 20% as test data. For the training data set, image data enhancement methods such as rotation ± 5 degrees, left and right translation, up and down translation, brightness random scaling with factor c ∈ [0.8, 1.2], horizontal flipping, etc. are used to expand the library to more than 8 times the original size. In terms of operations such as , translation, and horizontal flipping, the target image for foreground and background segmentation should also be transformed accordingly. Data augmentation can effectively avoid over-fitting during network training.
[0...
example 2
[0065] 1. Use the matting function module attached to "Light and Shadow Magic Hand" or the GrabCut+Lazy Snapping tool to interactively remove the background of the training and test sets of insect specimen images, and set the background to black and the foreground to white to obtain the front The target image for background segmentation.
[0066] 2. Randomly select 80% of the data set as training data, and the remaining 20% as test data. For the training data set, the image data enhancement methods such as rotation 5 degrees, left and right translation, up and down translation, brightness random scaling with factor c∈[0.8, 1.2], horizontal flipping, etc. are used to expand the library to more than 8 times the original size. In terms of translation and horizontal flip operations, the target image for foreground and background segmentation should also be transformed accordingly. Data augmentation can effectively avoid over-fitting during network training.
[0067] 3. Modify ...
PUM
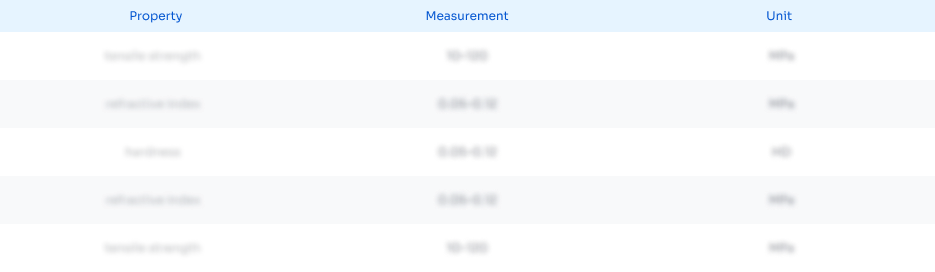
Abstract
Description
Claims
Application Information
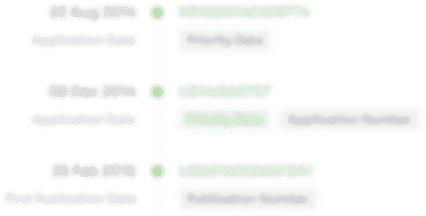
- R&D Engineer
- R&D Manager
- IP Professional
- Industry Leading Data Capabilities
- Powerful AI technology
- Patent DNA Extraction
Browse by: Latest US Patents, China's latest patents, Technical Efficacy Thesaurus, Application Domain, Technology Topic, Popular Technical Reports.
© 2024 PatSnap. All rights reserved.Legal|Privacy policy|Modern Slavery Act Transparency Statement|Sitemap|About US| Contact US: help@patsnap.com