Method for classifying encrypted traffic and server, and computer readable storage medium
A traffic classification and computer program technology, applied in the field of computer networks, can solve problems such as the limitation of message types, and achieve the effects of reducing sparsity, saving memory overhead, and expanding differences
- Summary
- Abstract
- Description
- Claims
- Application Information
AI Technical Summary
Problems solved by technology
Method used
Image
Examples
example 1
[0034] Example 1 Combining Logistic Regression Classifier to Classify 5 Applications
[0035] Classify encrypted traffic by Markov probabilistic fingerprints based on multidimensional attributes. From July 20, 2017, the traffic on a certain campus network was captured to passively mark the traffic of 5 software such as alicdn, JD, and onenote (a total of 19w+ streams, 119w+ data packets) as the labeled training data set. The application traffic extracts the message type sequence and packet length sequence. Then count the number of fields that appear in the message type to a total of 99, and encode the 99 different messagetype fields from 1 to 99 in sequence to form a message type encoded file. The number of representative lengths for each application covering 90% of the traffic is 320, respectively. 66, 141, 150 and 8. Use the message type encoding file to encode all message type sequences to form a message type Markov transition matrix corresponding to each application. Si...
example 2
[0036] Example 2 Combined with Random Forest Classifier to Classify 5 Applications
[0037] Classify encrypted traffic by Markov probabilistic fingerprints based on multidimensional attributes. From July 20, 2017, the traffic on a certain campus network was captured to passively mark the traffic of 5 software such as alicdn, JD, and onenote (a total of 19w+ streams, 119w+ data packets) as the labeled training data set. The application traffic extracts the message type sequence and packet length sequence. Then count the number of fields that appear in the message type to a total of 99, and encode the 99 different messagetype fields from 1 to 99 in sequence to form a message type encoded file (that is, encode the different message type fields that appear in sequence to generate a message type encoded file ), the number of representative lengths corresponding to 90% of traffic covered by each application is 320, 66, 141, 150 and 8 respectively. Use the message type encoding fil...
example 3
[0038] Example 3 Combining Random Forest Classifier to Classify 10 Applications
[0039] Classify encrypted traffic by Markov probabilistic fingerprints based on multidimensional attributes. From July 20, 2017, the traffic on a certain campus network was captured to passively mark the traffic of 10 applications such as github, QQ and baidu (a total of 35w+ streams, 241w+ data packets) as the marked training data set. The application traffic extracts the message type sequence and packet length sequence. Then count the number of fields that appear in the message type to a total of 117, encode the 117 different messagetype fields from 1 to 117 in sequence to form a message type encoding file, and obtain the corresponding representative length set. Use the message type encoding file to encode all message type sequences to form a message type Markov transition matrix corresponding to each application. Similarly, the original length sequence is transformed into a representative le...
PUM
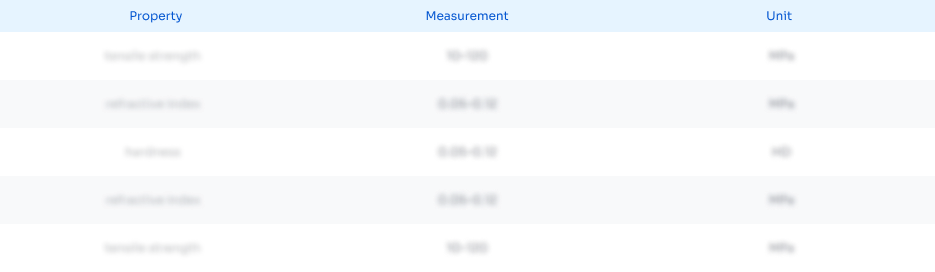
Abstract
Description
Claims
Application Information
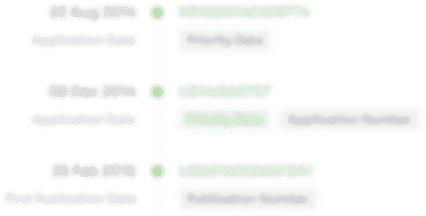
- R&D
- Intellectual Property
- Life Sciences
- Materials
- Tech Scout
- Unparalleled Data Quality
- Higher Quality Content
- 60% Fewer Hallucinations
Browse by: Latest US Patents, China's latest patents, Technical Efficacy Thesaurus, Application Domain, Technology Topic, Popular Technical Reports.
© 2025 PatSnap. All rights reserved.Legal|Privacy policy|Modern Slavery Act Transparency Statement|Sitemap|About US| Contact US: help@patsnap.com