Short-period forecast method for power load
A power load, short-term forecasting technology, applied in the direction of load forecasting, electrical components, circuit devices, etc. in the AC network, can solve the problems of inability to handle multi-variable ARIMA, easy to fall into local space, complex calculation process, etc., and achieve accurate forecast results. Reliable, lower electricity costs, and accurate prediction results
- Summary
- Abstract
- Description
- Claims
- Application Information
AI Technical Summary
Problems solved by technology
Method used
Image
Examples
Embodiment
[0032] Such as figure 1 As shown, a short-term electric load forecasting method, the method includes the following steps:
[0033] (1) Obtain the historical power load data sequence, and calculate the Hurst exponent H of the historical power load data sequence;
[0034] (2) Based on the Hurst exponent H, a fractional Brownian motion model for predicting electric load is established;
[0035] (3) Globally optimize the Hurst exponent H in the fractional Brownian motion model to obtain the optimal value H of the Hurst exponent gbest , and then get the fractional Brownian motion optimization model;
[0036] (4) Using fractional Brownian motion optimization model to predict power load data.
[0037] The Hurst exponent H of the power load data sequence in step (1) is obtained by the rescaled range analysis method (R / S method).
[0038] For the historical power load data sequence {y t ,t=0,1,2...n},y t Represents the historical power load data at time t, and its partial sum is:...
PUM
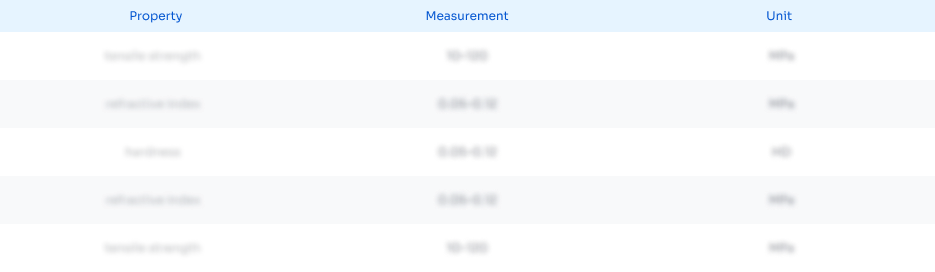
Abstract
Description
Claims
Application Information
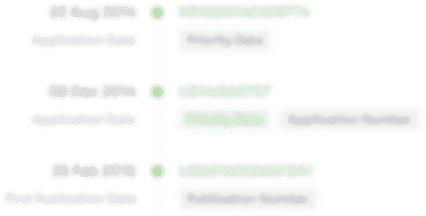
- R&D
- Intellectual Property
- Life Sciences
- Materials
- Tech Scout
- Unparalleled Data Quality
- Higher Quality Content
- 60% Fewer Hallucinations
Browse by: Latest US Patents, China's latest patents, Technical Efficacy Thesaurus, Application Domain, Technology Topic, Popular Technical Reports.
© 2025 PatSnap. All rights reserved.Legal|Privacy policy|Modern Slavery Act Transparency Statement|Sitemap|About US| Contact US: help@patsnap.com