Hyperspectral image lossless compression method based on deep learning
A hyperspectral image, lossless compression technology, applied in the field of image processing, can solve the problems of model generalization ability and prediction accuracy, insufficient information utilization, etc., to increase generalization ability, improve compression rate, and improve extraction The effect of a characteristic's ability
- Summary
- Abstract
- Description
- Claims
- Application Information
AI Technical Summary
Problems solved by technology
Method used
Image
Examples
Embodiment 1
[0024] At present, hyperspectral remote sensing technology is at the forefront of remote sensing technology, and a lot of manpower and material resources have been invested at home and abroad to study it, but there are still huge difficulties in the real-time transmission of data acquired by hyperspectral imagers. In response to this problem, the present invention conducts research from the perspective of image lossless compression, focuses on more efficient lossless compression methods, and proposes a hyperspectral image lossless compression method based on deep learning, see figure 1 , including the following steps:
[0025] (1) Clustering all spectral lines in the original hyperspectral image: input the hyperspectral image to be compressed, which has M bands. The standard deviation of hyperspectral image data bands is large and the amount of information is rich; while the correlation coefficient between bands is small and the redundancy of band information is small. In this...
Embodiment 2
[0032] The hyperspectral image lossless compression method based on deep learning is the same as embodiment 1, and the spectral line after clustering described in step (2) is predicted using a deep recurrent neural network, including the following steps:
[0033] (2a) Intra-spectrum prediction is used for the first band, that is, adjacent pixel prediction is used for intra-band prediction, that is, the pixel value of the first band of the first spectral line is directly encoded and transmitted as the residual, and the pixel value of the second spectral line is directly encoded and transmitted. A band is predicted from the first spectral line, and in this way the first bands of all spectral lines are obtained. The adjacent pixel prediction used in this example is to use the core algorithm LOCO-I of the JPEG-LS compression standard to perform intra-band prediction. The formula is
[0034]
[0035] (2b) From the second wave band, the recurrent neural network is used to carry o...
Embodiment 3
[0041] The hyperspectral image lossless compression method based on deep learning is the same as embodiment 1-2, the network structure of the recurrent neural network described in step (2b), and the structure of the neural network is shown in the appendix image 3 As shown, it includes the following parts in order from input to output: 1 input layer, 3 hidden layers, 1 fully connected layer, and an output layer, using softmax for classification. In this example, an expanded network is used. The input layer has 20 units, and each hidden layer has 40 units. The fully connected layer after the hidden layer fuses the features extracted from the hidden layer to obtain a unit in the output layer.
[0042] Compared with the traditional method, the present invention has more efficient learning efficiency. The hidden layer uses an expanded network, that is, the number of units used in the hidden layer is input to the layer, which increases the ability of the model to extract features and ...
PUM
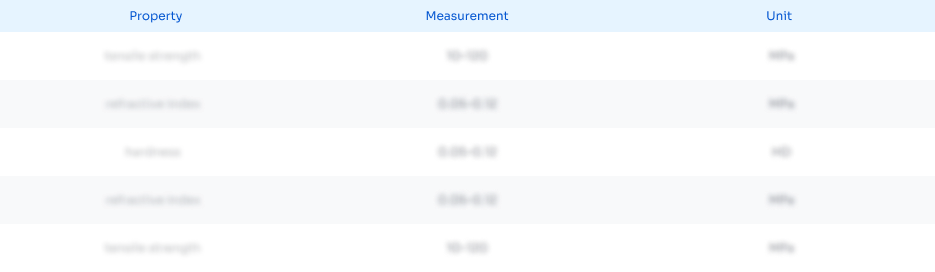
Abstract
Description
Claims
Application Information
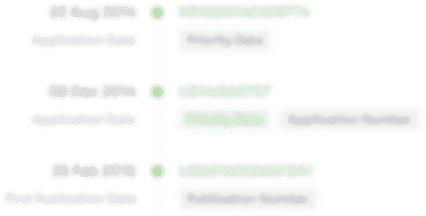
- R&D Engineer
- R&D Manager
- IP Professional
- Industry Leading Data Capabilities
- Powerful AI technology
- Patent DNA Extraction
Browse by: Latest US Patents, China's latest patents, Technical Efficacy Thesaurus, Application Domain, Technology Topic, Popular Technical Reports.
© 2024 PatSnap. All rights reserved.Legal|Privacy policy|Modern Slavery Act Transparency Statement|Sitemap|About US| Contact US: help@patsnap.com