Mechanical multi-fault diagnosis method based on signal atomic-driven wavelet reproducing kernel machine learning
A technology of machine learning and diagnostic methods, which is applied in the testing of machines/structural components, biological models, testing of mechanical components, etc. It can solve problems such as unformed perfect theories, achieve strong practicability and improve computational efficiency
- Summary
- Abstract
- Description
- Claims
- Application Information
AI Technical Summary
Problems solved by technology
Method used
Image
Examples
Embodiment 1
[0066] The specific parameters of the test planetary gearbox are shown in Table 1:
[0067] Table 1 Specific parameters of planetary gearbox
[0068]
[0069] During the test, the motor drives the test bearing to rotate, the motor rotation frequency is 30Hz, and the signal sampling frequency is 10kHz. The types of faults where the faulty gear is a sun gear include: root crack faults, tooth surface wear faults, broken teeth faults, and missing teeth faults.
[0070] Step 1: Add labels 1, 2, 3, and 4 to the four types of sun gear failures; take 4096 points as the data length, and intercept 50 sets of data for each state ( Figure 3-Figure 6 are the time-domain waveforms corresponding to the four kinds of faults). Therefore, for a total of 200 sets of data in 4 states for fault pattern recognition, 60% of the samples are used to train the SVM diagnostic model of RBF kernel function and multi-scale regeneration kernel function, and the remaining 40% of the samples are used fo...
PUM
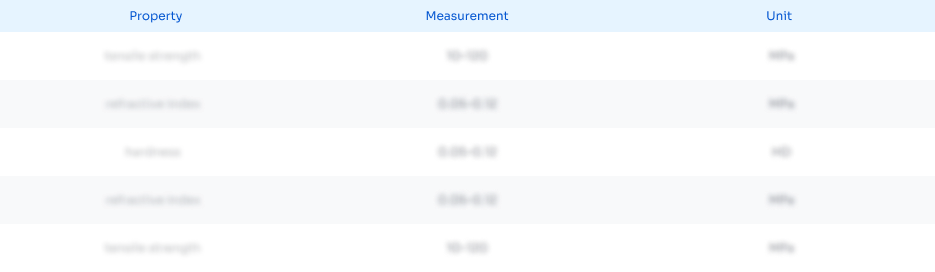
Abstract
Description
Claims
Application Information
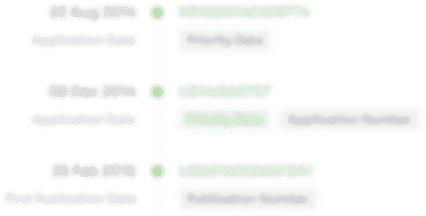
- R&D
- Intellectual Property
- Life Sciences
- Materials
- Tech Scout
- Unparalleled Data Quality
- Higher Quality Content
- 60% Fewer Hallucinations
Browse by: Latest US Patents, China's latest patents, Technical Efficacy Thesaurus, Application Domain, Technology Topic, Popular Technical Reports.
© 2025 PatSnap. All rights reserved.Legal|Privacy policy|Modern Slavery Act Transparency Statement|Sitemap|About US| Contact US: help@patsnap.com