An unsupervised/semi-supervised CT image reconstruction depth network train method
A CT image and deep network technology, applied in the field of image processing, can solve the problems of high computational cost, lack of extraction of common features, influence of network accuracy, etc., to avoid the acquisition process, increase the practicability, and achieve the effects of high imaging quality.
- Summary
- Abstract
- Description
- Claims
- Application Information
AI Technical Summary
Problems solved by technology
Method used
Image
Examples
Embodiment 1
[0057] The real patient CT data provided by the "2016Low-dose CT Grand Challenge datasets" website is adopted as the experimental data source of the present invention. Among them, we only use 50 low-dose CT data with a dose of 10mAs as our experimental training data (excluding prediction data). Choose the second-order TV sparsity property of the chord graph (such as Figure 5 shown) is a priori, refer to figure 1 , the present invention comprises the steps in turn:
[0058] Step S1: Obtain unlabeled low-dose projection data under the CT scanning protocol (50 pieces of low-dose CT chord diagram data with a dose of 10mAs are used here), and initialize the network structure and network parameters;
[0059] Step S2: Skip this step due to lack of labeled data;
[0060] Step S3: Determine the loss function corresponding to the unlabeled data, the expression is:
[0061]
[0062] where θ is the network parameter, f θ (x) is the CT chord diagram data output by the network, p i...
Embodiment 2
[0088] The real patient CT data provided by the "2016Low-dose CT Grand Challenge datasets" website is adopted as the experimental data source of the present invention. Among them, we use 50 low-dose CT data with a dose of 10mAs, and 10 pairs of CT chord diagram data pairs with a dose of (10mAs, 200mAs) as our experimental training data (excluding prediction data). Choose the second-order TV sparsity property of the chord graph (such as Figure 5 shown) is a priori, refer to figure 1 , the present invention comprises the steps successively:
[0089] Step S1: Obtain unlabeled low-dose CT chord data under the CT scanning protocol (50 low-dose CT data with a dose of 10mAs, 10 pairs of CT chord data with a dose of (10mAs, 200mAs) are used here), and initialize the network structure and network parameters;
[0090] Step S2: Determine the loss function corresponding to the labeled data, the expression is:
[0091] loss=‖f θ (x)-y true ‖ 2 (1)
[0092] Step S3: Determine the ...
PUM
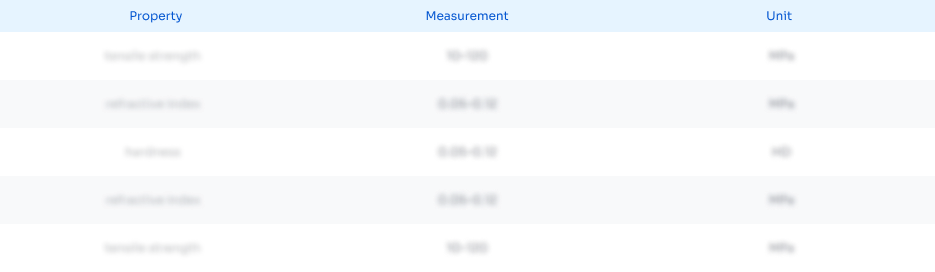
Abstract
Description
Claims
Application Information
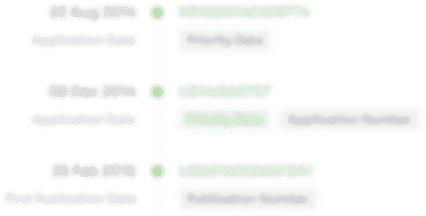
- Generate Ideas
- Intellectual Property
- Life Sciences
- Materials
- Tech Scout
- Unparalleled Data Quality
- Higher Quality Content
- 60% Fewer Hallucinations
Browse by: Latest US Patents, China's latest patents, Technical Efficacy Thesaurus, Application Domain, Technology Topic, Popular Technical Reports.
© 2025 PatSnap. All rights reserved.Legal|Privacy policy|Modern Slavery Act Transparency Statement|Sitemap|About US| Contact US: help@patsnap.com