DBN (deep belief network)-GA neural network-based fault detection method of high-voltage circuit breaker
A high-voltage circuit breaker and fault detection technology, applied in circuit breaker testing, instruments, measuring electricity, etc., can solve the problems of single learning process and incomplete training, so as to make up for insufficient detection, reduce training time, and accurately determine fault types. Effect
- Summary
- Abstract
- Description
- Claims
- Application Information
AI Technical Summary
Problems solved by technology
Method used
Image
Examples
Embodiment
[0123] Take t0 as the zero point of the command time to extract the fault characteristic parameters I1, I2, I3, t1, t2, t3, t4, t5 to monitor the state of the circuit breaker, and obtain ten sets of fault sample data. These ten sets of fault sample data include normal mechanism ( A), the operating voltage is too low (B), the closing iron core is jammed at the beginning (C), the operating mechanism is jammed (D), and the empty travel of the closing iron core is too large (E). The data collection conditions are shown in Table 1 shown;
[0124] Table 1 Fault sample data
[0125]
[0126] The characteristic curve of closing / opening coil current is as follows: Figure 4 As shown, it can be seen that:
[0127] (1) Phase I, t=t0~t1; the coil starts to be energized at t0, and the iron core starts to move at t1; t0 is the moment when the circuit breaker opens and closes the command, and it is the starting point of the circuit breaker’s opening and closing action timing; T1 is The...
PUM
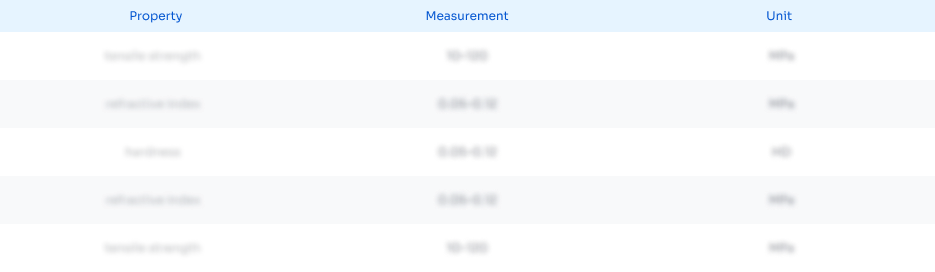
Abstract
Description
Claims
Application Information
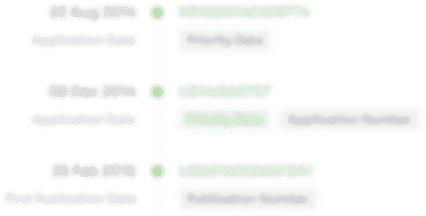
- R&D Engineer
- R&D Manager
- IP Professional
- Industry Leading Data Capabilities
- Powerful AI technology
- Patent DNA Extraction
Browse by: Latest US Patents, China's latest patents, Technical Efficacy Thesaurus, Application Domain, Technology Topic, Popular Technical Reports.
© 2024 PatSnap. All rights reserved.Legal|Privacy policy|Modern Slavery Act Transparency Statement|Sitemap|About US| Contact US: help@patsnap.com