Hardness Prediction Algorithm of Ferromagnetic Material Based on Improved Algorithm of BP Neural Network
A BP neural network and ferromagnetic material technology, applied in the field of non-destructive testing algorithms for ferromagnetic materials, can solve problems such as large errors in hardness prediction results, damage to the performance of the test object, and inability to conduct destructive tests, and achieve anti-interference performance. strong effect
- Summary
- Abstract
- Description
- Claims
- Application Information
AI Technical Summary
Problems solved by technology
Method used
Image
Examples
Embodiment Construction
[0024] Embodiments of the present invention are described in detail below, examples of which are shown in the drawings, wherein the same or similar reference numerals designate the same or similar elements or elements having the same or similar functions throughout. The embodiments described below by referring to the figures are exemplary only for explaining the present invention and should not be construed as limiting the present invention.
[0025] Such as figure 1 As shown, according to the ferromagnetic material hardness prediction algorithm based on the BP neural network improved algorithm of the present invention, the existing ferromagnetic material is subjected to hardness non-destructive testing, and the specific implementation steps are as follows:
[0026] Step 1: Collect the Barkhausen signal of the ferromagnetic material, divide the signal set, and obtain the Barkhausen noise training set and the Barkhausen noise test set. There are 720 samples in the training set...
PUM
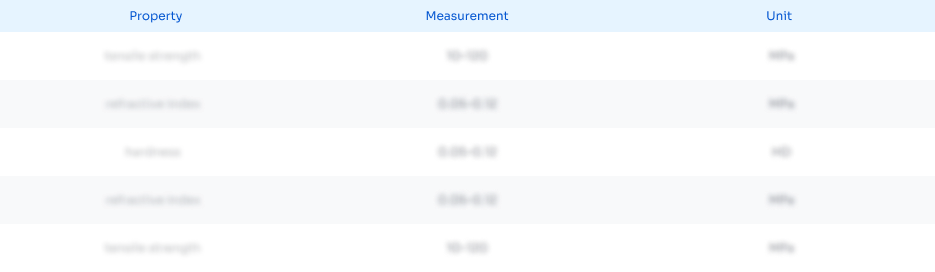
Abstract
Description
Claims
Application Information
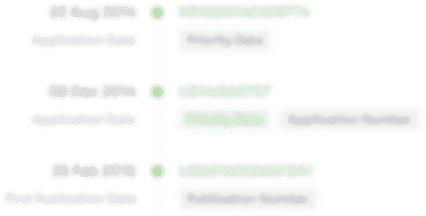
- R&D
- Intellectual Property
- Life Sciences
- Materials
- Tech Scout
- Unparalleled Data Quality
- Higher Quality Content
- 60% Fewer Hallucinations
Browse by: Latest US Patents, China's latest patents, Technical Efficacy Thesaurus, Application Domain, Technology Topic, Popular Technical Reports.
© 2025 PatSnap. All rights reserved.Legal|Privacy policy|Modern Slavery Act Transparency Statement|Sitemap|About US| Contact US: help@patsnap.com