A visual defect detection method based on a multi-spectral depth convolution neural network
A neural network, visual defect technology, applied in image data processing, instrument, character and pattern recognition, etc., to achieve the effect of improving separation ability, strong adaptability, and strengthening extraction ability
- Summary
- Abstract
- Description
- Claims
- Application Information
AI Technical Summary
Problems solved by technology
Method used
Image
Examples
Embodiment 1
[0060] This embodiment is based on the visual defect detection method of the multi-spectral deep convolutional neural network, and the method includes three step units:
[0061] The first step, defect feature analysis and data set:
[0062] 1-1 Image acquisition: use a color camera to collect images, remove the background to obtain a color picture, and the color picture is used as the original data set;
[0063] 1-2 Feature analysis: analyze and observe the characteristics of solar surface defects in different spectra, and obtain the feature maps of chipping, thick lines, broken grids, scratches, slurry leakage, color difference, and dirty surface defects;
[0064] 1-3 Image cutting: on the basis of step 1-2, use the sliding segmentation method to divide the original data set in step 1-1 into small pictures, and use the small picture as the target image;
[0065] The picture size of described original data set is 1868 * 1868; Small picture refers to the picture of 469 * 469 s...
PUM
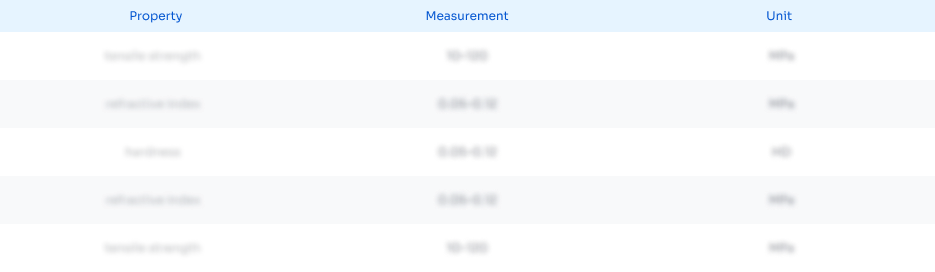
Abstract
Description
Claims
Application Information
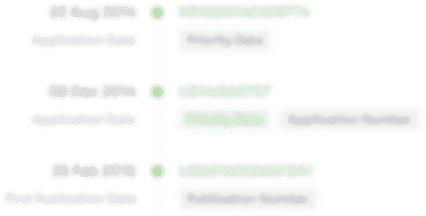
- R&D
- Intellectual Property
- Life Sciences
- Materials
- Tech Scout
- Unparalleled Data Quality
- Higher Quality Content
- 60% Fewer Hallucinations
Browse by: Latest US Patents, China's latest patents, Technical Efficacy Thesaurus, Application Domain, Technology Topic, Popular Technical Reports.
© 2025 PatSnap. All rights reserved.Legal|Privacy policy|Modern Slavery Act Transparency Statement|Sitemap|About US| Contact US: help@patsnap.com