An MDS-based prediction model of RBF for petrochemical industry production capacity
A prediction model and production capacity technology, applied in biological neural network models, prediction, energy industry and other directions, can solve the problems of incompleteness and inaccurate prediction results, and achieve the effect of effective prediction, improving energy efficiency and solving inaccuracy
- Summary
- Abstract
- Description
- Claims
- Application Information
AI Technical Summary
Problems solved by technology
Method used
Image
Examples
Embodiment 1
[0059] In order to better predict the energy efficiency of the petrochemical industry, this embodiment proposes an MDS-based RBF prediction model for the production capacity of the petrochemical industry. In this embodiment, the category of raw materials can be automatically obtained in the low-dimensional space, and the distance between the original data in the high-dimensional space is maintained, and then analyzed and selected in the low-dimensional space as the training set and test set of RBF to predict ethylene production.
[0060] figure 1 It is a flow chart of ethylene production provided by Example 1 of the present invention. Such as figure 1As shown, this embodiment uses a dimensionality reduction method of multidimensional scaling (MDS) to reduce the original data from a high-dimensional space to a low-dimensional space and make it possible to maintain the distance in the high-dimensional space, so that the low-dimensional space can In the analysis and selection ...
PUM
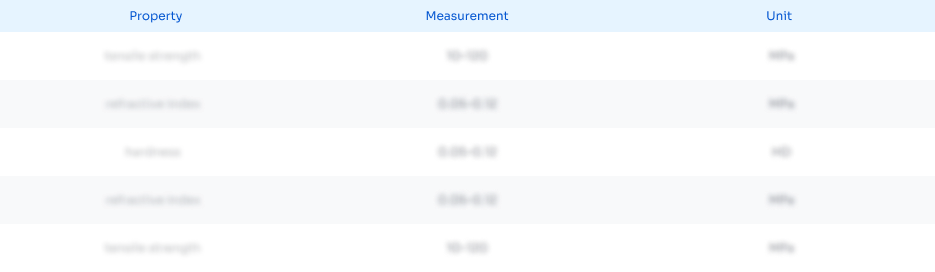
Abstract
Description
Claims
Application Information
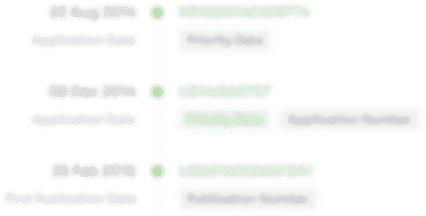
- R&D Engineer
- R&D Manager
- IP Professional
- Industry Leading Data Capabilities
- Powerful AI technology
- Patent DNA Extraction
Browse by: Latest US Patents, China's latest patents, Technical Efficacy Thesaurus, Application Domain, Technology Topic, Popular Technical Reports.
© 2024 PatSnap. All rights reserved.Legal|Privacy policy|Modern Slavery Act Transparency Statement|Sitemap|About US| Contact US: help@patsnap.com