Wind power prediction method based on a deep convolutional neural network
A technology of wind power prediction and deep convolution, applied in biological neural network models, predictions, neural architectures, etc., can solve problems such as increased computational costs, inability to express wind spatiotemporal changes, and limited wind power prediction, etc., to achieve extended expression capabilities Effect
- Summary
- Abstract
- Description
- Claims
- Application Information
AI Technical Summary
Problems solved by technology
Method used
Image
Examples
Embodiment 1
[0045] An embodiment of the present invention provides a wind power prediction method based on a deep convolutional neural network, see figure 1 , the method includes:
[0046] 101: Select and collect wind farm data, and use the grid space embedding method to map the real coordinates of the wind turbine to the plane grid;
[0047] Wherein, the grid space embedding method is well known to those skilled in the art, and the embodiment of the present invention does not repeat it here.
[0048] The steps of selecting and collecting wind farm data are specifically: selecting a wind farm area within a certain range of latitude and longitude, and collecting historical data of all (a total of n) wind turbines in the wind farm area, with a fixed time interval. Based on the above data, predict the wind power output of the wind turbine after a period of time.
[0049] Among them, using the grid space embedding method to map the real coordinates of the wind turbine to the plane grid, the...
Embodiment 2
[0060] Combine below Figure 2-Figure 4 The scheme in Example 1 is further introduced, see the following description for details:
[0061] The embodiment of the present invention proposes STF to represent the information of the state of the wind farm, and proposes three STF-based deep convolutional neural network models to accurately and efficiently predict wind power, such as figure 1 As shown, it is an overall schematic diagram of a specific embodiment of wind power prediction using a deep convolutional neural network model in an embodiment of the present invention, including:
[0062] 201: Select the longitude range as x 1 ~x 2 , the dimension range is y 1 ~y 2 wind farm area, and collect historical data such as wind speed and power of n wind turbines in the wind farm area, with a fixed time interval;
[0063] 202: Preprocessing the data collected in step 201 to form continuous time series historical data for each wind turbine in the wind farm;
[0064] Wherein, based...
Embodiment 3
[0089] Combined with the calculation formula below, Figure 5 , and table 1 carries out feasibility verification to the scheme in embodiment 1 and 2, see the following description for details:
[0090] Accuracy is the most important aspect to measure the effect of wind power forecasting, and the main indicators for evaluating accuracy are mean square error (MSE) and root square error (RMSE). Wherein, RMSE is the arithmetic square root of MSE, so the embodiment of the present invention selects MSE as the evaluation standard of wind power prediction. The calculation method of MSE is shown in formula (1), where real is the real value sequence, predictions is the predicted value sequence, and n is the sequence length.
[0091]
[0092] The MSE is calculated for the prediction results of each model, and the prediction effects of the three models are compared and analyzed, and then the advantages and disadvantages of the models, the temporal and spatial characteristics of wind p...
PUM
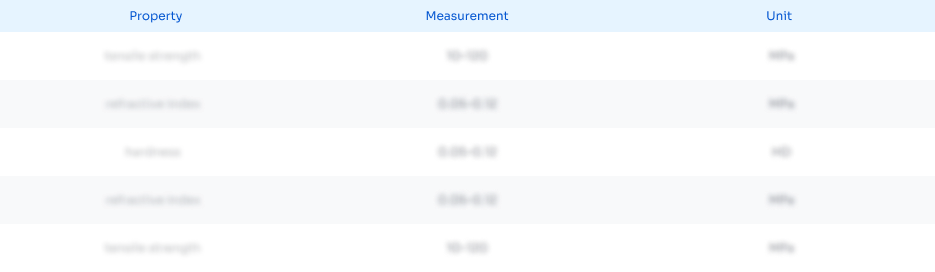
Abstract
Description
Claims
Application Information
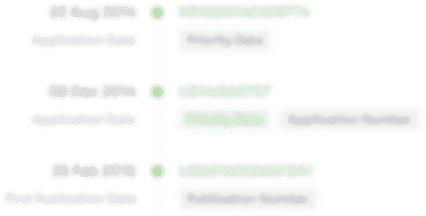
- R&D Engineer
- R&D Manager
- IP Professional
- Industry Leading Data Capabilities
- Powerful AI technology
- Patent DNA Extraction
Browse by: Latest US Patents, China's latest patents, Technical Efficacy Thesaurus, Application Domain, Technology Topic.
© 2024 PatSnap. All rights reserved.Legal|Privacy policy|Modern Slavery Act Transparency Statement|Sitemap