A perceptron network data classification method based on an AdaBoost algorithm
A technology of network data and classification methods, applied in the direction of instruments, computing, computer parts, etc., can solve the problems of costing a lot of money and time, and achieve the effect of strong efficiency, improved classification accuracy, and simple model structure.
- Summary
- Abstract
- Description
- Claims
- Application Information
AI Technical Summary
Problems solved by technology
Method used
Image
Examples
Embodiment Construction
[0035] specific implementation plan
[0036] The present invention will be described in further detail below through examples of implementation.
[0037] Taking the fault diagnosis of refrigerator charging as an example, the selected data set comes from a multi-line refrigeration charging experiment, and the equipment consists of 3 indoor units and 1 outdoor unit. The data set includes a total of 18,000 data records, and the data includes 22 characteristic variables such as outdoor ambient temperature, local machine distribution capacity, compressor operating frequency, fan operating frequency, compressor shell top temperature, and compressor exhaust temperature. There are 3 types of failure states: insufficient refrigerant charge, normal refrigerant charge and excessive refrigerant charge. The data set is divided into two parts, the training set and the test set. The number of samples in the training set is 14400, and the number of samples in the test set is 3600.
[0038] ...
PUM
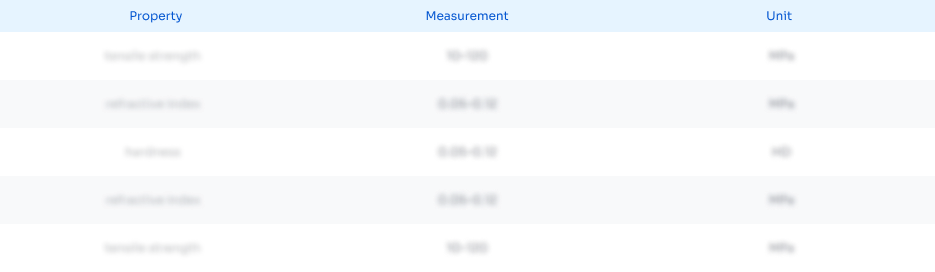
Abstract
Description
Claims
Application Information
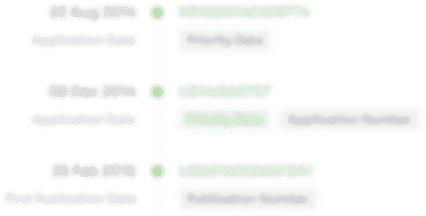
- Generate Ideas
- Intellectual Property
- Life Sciences
- Materials
- Tech Scout
- Unparalleled Data Quality
- Higher Quality Content
- 60% Fewer Hallucinations
Browse by: Latest US Patents, China's latest patents, Technical Efficacy Thesaurus, Application Domain, Technology Topic, Popular Technical Reports.
© 2025 PatSnap. All rights reserved.Legal|Privacy policy|Modern Slavery Act Transparency Statement|Sitemap|About US| Contact US: help@patsnap.com