Image data expansion method for deep learning model training and learning
An image data and model training technology, applied in image data processing, image analysis, character and pattern recognition, etc., can solve the problems of insufficient image data heterogeneity, insufficient data volume, unfavorable image analysis of rare cases, etc. The effect of overfitting, reducing the burden on doctors, improving accuracy and generalization ability
- Summary
- Abstract
- Description
- Claims
- Application Information
AI Technical Summary
Problems solved by technology
Method used
Image
Examples
Embodiment 1
[0030] Example 1: as Figure 1-6 As shown, this image image data expansion method for deep learning model training and learning includes the following steps:
[0031] (1) Determine the data type and identify CT or MRI image data;
[0032](2) For the image data, determine whether there is a defined ROI, and combine the size of the tumor area, select the corresponding method to complete the construction of the image data set;
[0033] (3) Use the basic image transformation method to train the image data set to obtain a preliminary training data set. Obtain a preliminary training data set, and the initially expanded data set has enabled the deep learning network to achieve a certain performance increase. At this time, for the data after the initial expansion, a further richer data expansion can be performed using a Generative Adversarial Network (GAN). After that, the data set can be sent to the deep learning network model for parameter training. In the parameter training pro...
Embodiment 2
[0045] Embodiment 2: as Figure 1-6 As shown, the video image data expansion method for deep learning model training and learning includes the following steps:
[0046] (1) Determine the data type and identify CT or MRI image data;
[0047] (2) For the image data, judge whether there is a defined ROI, and combine the size of the tumor area, select the corresponding method to complete the construction of the image data set; that is, use the MRI data expansion method based on ROI, first select the region of interest in the original MRI image , and then use conventional methods to sequentially perform pruning, translation transformation, rotation, noise removal, resampling, mirror flip, reflection transformation, PCA dithering, and color dithering on the image of the region of interest to obtain the MR image dataset.
[0048] Data augmentation based on ROI is to randomly select blocks in the image data set of a given Region of Interest (ROI) derived by existing algorithms, that ...
Embodiment 3
[0054] Embodiment 3: as Figure 1-6 As shown, the video image data expansion method for deep learning model training and learning includes the following steps:
[0055] (1) Determine the data type and identify CT or MRI image data;
[0056] (2) For the image data, judge whether there is a defined ROI, and combine the size of the tumor area, select the corresponding method to complete the construction of the image data set; that is, use the MRI data expansion method based on ROI, first select the region of interest in the original MRI image , and then use conventional methods to sequentially perform pruning, translation transformation, rotation, noise removal, resampling, mirror flip, reflection transformation, PCA dithering, and color dithering on the image of the region of interest to obtain the MR image dataset.
[0057] Data augmentation based on ROI is to randomly select blocks in the image data set of a given Region of Interest (ROI) derived by existing algorithms, that ...
PUM
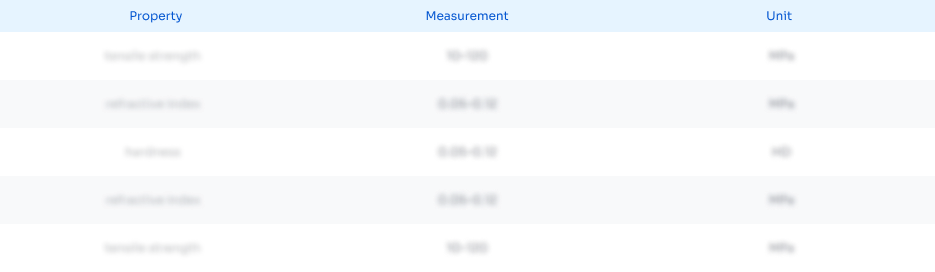
Abstract
Description
Claims
Application Information
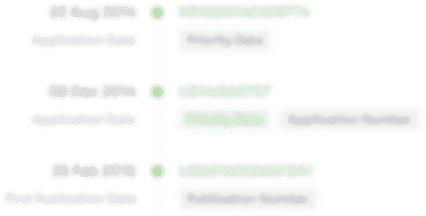
- R&D
- Intellectual Property
- Life Sciences
- Materials
- Tech Scout
- Unparalleled Data Quality
- Higher Quality Content
- 60% Fewer Hallucinations
Browse by: Latest US Patents, China's latest patents, Technical Efficacy Thesaurus, Application Domain, Technology Topic, Popular Technical Reports.
© 2025 PatSnap. All rights reserved.Legal|Privacy policy|Modern Slavery Act Transparency Statement|Sitemap|About US| Contact US: help@patsnap.com