Rolling bearing remaining life prediction method based on long-short term memory network
A long-short-term memory and rolling bearing technology, which is applied in mechanical bearing testing, neural learning methods, biological neural network models, etc., can solve problems such as long-term dependence of time series, achieve long-term dependence problems, wide application prospects, and flexible parameter adjustment Effect
- Summary
- Abstract
- Description
- Claims
- Application Information
AI Technical Summary
Problems solved by technology
Method used
Image
Examples
Embodiment Construction
[0029] The method for predicting the remaining life of a rolling bearing based on a long-short-term memory network of the present invention will be described in more detail below in conjunction with a schematic diagram, wherein a preferred embodiment of the present invention is shown, and it should be understood that those skilled in the art can modify the present invention described here, and The advantageous effects of the invention are still achieved. Therefore, the following description should be understood as the broad knowledge of those skilled in the art, but not as a limitation of the present invention.
[0030] Such as figure 1 As shown, a method for predicting the remaining life of a rolling bearing based on a long-short-term memory network, including steps S1 to S7, is as follows:
[0031] S1: Extract the characteristics of six rolling bearing wear signals, including root mean square value, absolute mean value, average frequency, center frequency, root mean square ...
PUM
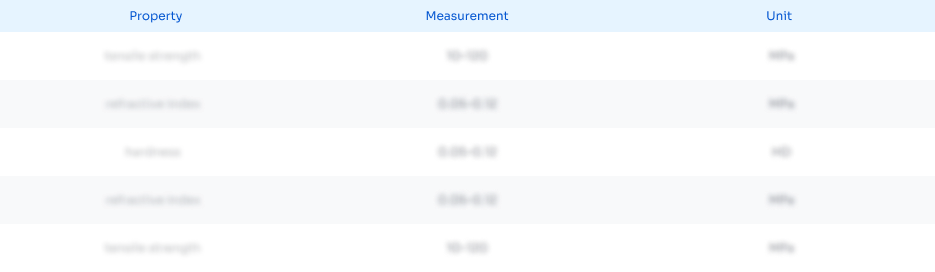
Abstract
Description
Claims
Application Information
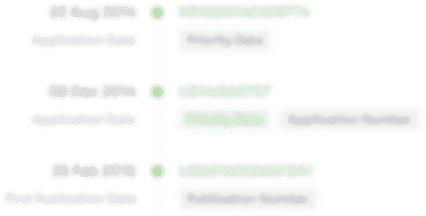
- R&D Engineer
- R&D Manager
- IP Professional
- Industry Leading Data Capabilities
- Powerful AI technology
- Patent DNA Extraction
Browse by: Latest US Patents, China's latest patents, Technical Efficacy Thesaurus, Application Domain, Technology Topic.
© 2024 PatSnap. All rights reserved.Legal|Privacy policy|Modern Slavery Act Transparency Statement|Sitemap