Electric vehicle speed reducer remaining service life prediction method based on LSTM
A technology for automobile reducer and life prediction, which is applied in neural learning methods, special data processing applications, biological neural network models, etc., and can solve problems such as long-term dependence of time series
- Summary
- Abstract
- Description
- Claims
- Application Information
AI Technical Summary
Problems solved by technology
Method used
Image
Examples
Embodiment Construction
[0033] The present invention will be further described below in conjunction with the accompanying drawings.
[0034] Figure 1 to Figure 5 , an LSTM-based method for predicting the remaining service life of electric vehicle reducers, including the following steps:
[0035] S1: The construction of the degradation characteristic data set of the electric vehicle reducer, the process is as follows: extract the characteristics of 9 electric vehicle reducer degradation signals, including the root mean square value, standard deviation, and variance in the time domain; the root mean square value in the frequency domain , average frequency, center of gravity frequency; the energy spectrum of the fourth frequency band of the wavelet packet, the energy spectrum of the sixth frequency band of the wavelet packet, and the energy spectrum of the first EMD component in the time-frequency domain, such as Figure 4 shown.
[0036] S2: Normalize the characteristic data so that its characterist...
PUM
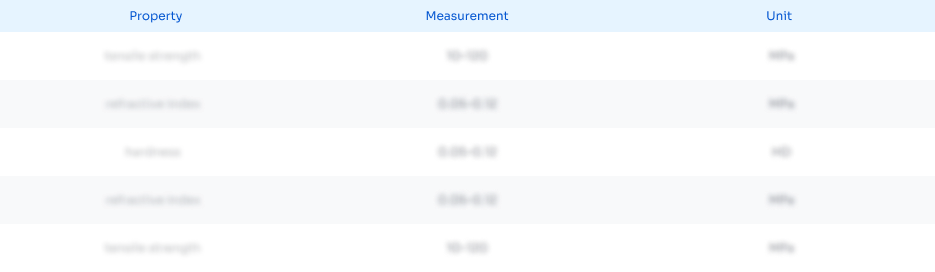
Abstract
Description
Claims
Application Information
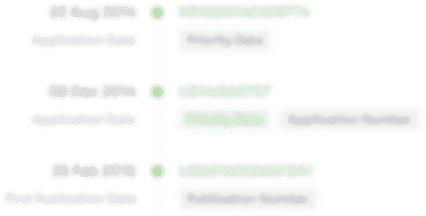
- Generate Ideas
- Intellectual Property
- Life Sciences
- Materials
- Tech Scout
- Unparalleled Data Quality
- Higher Quality Content
- 60% Fewer Hallucinations
Browse by: Latest US Patents, China's latest patents, Technical Efficacy Thesaurus, Application Domain, Technology Topic, Popular Technical Reports.
© 2025 PatSnap. All rights reserved.Legal|Privacy policy|Modern Slavery Act Transparency Statement|Sitemap|About US| Contact US: help@patsnap.com