Human Complex Behavior Recognition Method Based on Multi-Feature Fusion CNN-BLSTM
A multi-feature fusion and recognition method technology, applied in neural learning methods, character and pattern recognition, instruments, etc., can solve the problems of gradient dissipation, inability to fully extract time series data features, gradient explosion, etc., to improve the recognition accuracy, overcome the Effects of long-term dependency problems
- Summary
- Abstract
- Description
- Claims
- Application Information
AI Technical Summary
Problems solved by technology
Method used
Image
Examples
Embodiment Construction
[0029] The present invention will be further described below in conjunction with drawings and embodiments.
[0030] refer to Figure 1 ~ Figure 4 , a complex human behavior recognition method based on multi-feature fusion CNN-BLSTM, comprising the following steps:
[0031] Step 1, segmenting continuous sensor data;
[0032] The continuous sensor data is segmented through the sliding window. The process is: select a window size of 200 for 50% data overlapping segmentation. The window size of 200 means that the sensor frequency is 50 Hz, and a total of 4 seconds is collected as an input data. 50% data overlap refers to repeated segmentation, each window size is 200 current input data, and the last 100 data are used as the first 100 data of the next input data.
[0033] Step 2, extracting and selecting features of the segmented sensor data;
[0034] Extract features from the segmented sensor data and perform standardized processing. Through the feature selection algorithm, thi...
PUM
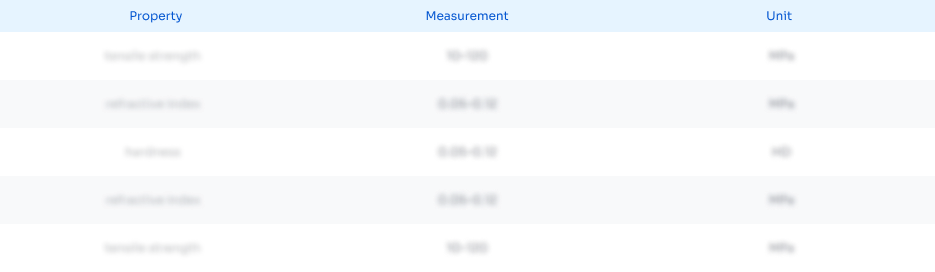
Abstract
Description
Claims
Application Information
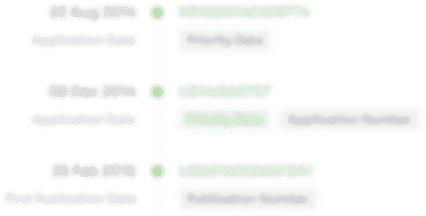
- R&D Engineer
- R&D Manager
- IP Professional
- Industry Leading Data Capabilities
- Powerful AI technology
- Patent DNA Extraction
Browse by: Latest US Patents, China's latest patents, Technical Efficacy Thesaurus, Application Domain, Technology Topic, Popular Technical Reports.
© 2024 PatSnap. All rights reserved.Legal|Privacy policy|Modern Slavery Act Transparency Statement|Sitemap|About US| Contact US: help@patsnap.com