A debris flow prediction method based on PCA and a mixed kernel function LSSVR
A hybrid kernel function and prediction method technology, applied in prediction, data processing applications, electrical digital data processing, etc., can solve problems such as low accuracy, model training performance defects, and prediction dimensionality disasters
- Summary
- Abstract
- Description
- Claims
- Application Information
AI Technical Summary
Problems solved by technology
Method used
Image
Examples
Embodiment
[0171] The experimental verification was carried out with the monitoring data of Mozigou, Taiping Village, Chengguan Town, Ziyang County, Ankang City, Shaanxi Province, respectively, a1: daily rainfall (mm), a2: infrasound (Hz), a3: mud level (mA), a4: soil Moisture content (%), a5: pore water pressure (KPa), a6: slope (°), a7: relative height difference (m) 7 parameters are used as the initial evaluation influencing factors, and the probability of debris flow is used as the prediction object, and the establishment A prediction model between the occurrence probability of debris flow and its impact factors. The 90 sets of original data were screened by the principal component analysis method for the characteristics of the principal component influencing factors. The variables extracted by PCA are as follows Figure 5 Shown, and the results of the kernel principal component matrix are shown in Table 2.
[0172] Depend on Figure 5 It can be seen that the cumulative contribut...
PUM
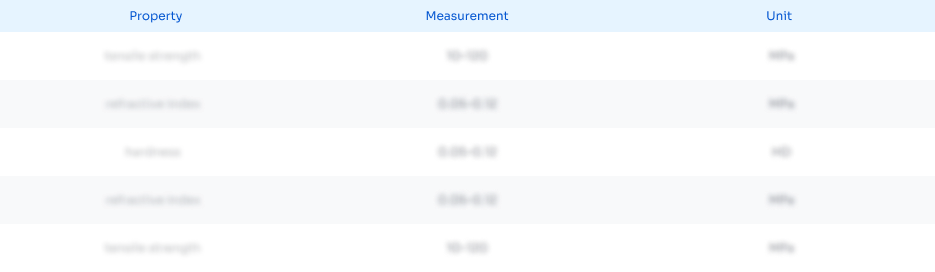
Abstract
Description
Claims
Application Information
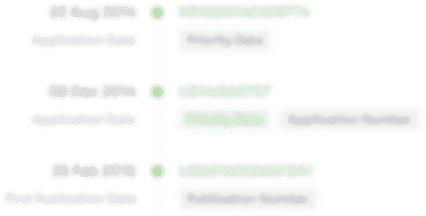
- R&D Engineer
- R&D Manager
- IP Professional
- Industry Leading Data Capabilities
- Powerful AI technology
- Patent DNA Extraction
Browse by: Latest US Patents, China's latest patents, Technical Efficacy Thesaurus, Application Domain, Technology Topic, Popular Technical Reports.
© 2024 PatSnap. All rights reserved.Legal|Privacy policy|Modern Slavery Act Transparency Statement|Sitemap|About US| Contact US: help@patsnap.com