Unmanned aerial vehicle image change detection method based on semantic segmentation and twin neural network
An image change detection and neural network technology, applied in the field of multi-temporal UAV image change detection, can solve the problems of loss detection accuracy, low feature robustness, and failure to consider multi-scale, etc., to improve quality and robustness Rodness, improve detection accuracy, and solve the effect of noise sensitivity
- Summary
- Abstract
- Description
- Claims
- Application Information
AI Technical Summary
Problems solved by technology
Method used
Image
Examples
Embodiment 1
[0051] The system environment of the present invention is Linux, using the Python language, and the deep learning framework used is Pytorch, which can efficiently train and test the neural network. For the block diagram of the simulation system of the present invention, refer to image 3 ;like figure 1 As shown, the specific implementation is as follows:
[0052] Step 1. Expand the dataset and divide the dataset:
[0053] S11. Expanding the dataset through data enhancement: the data enhancement methods used include operations such as flipping, changing contrast, changing brightness, sharpening, and intercepting;
[0054] S12. Divide the data set: divide the data set into the training set and the test set according to the ratio of 7:3.
[0055] Step 2. Build a twin neural network based on DeeplabV3:
[0056] S21. Use the AID dataset and the transfer learning method to fine-tune the ResNet50 network and build it as a ResNet-AID network;
[0057] S22. Using ResNet-AID as the ...
PUM
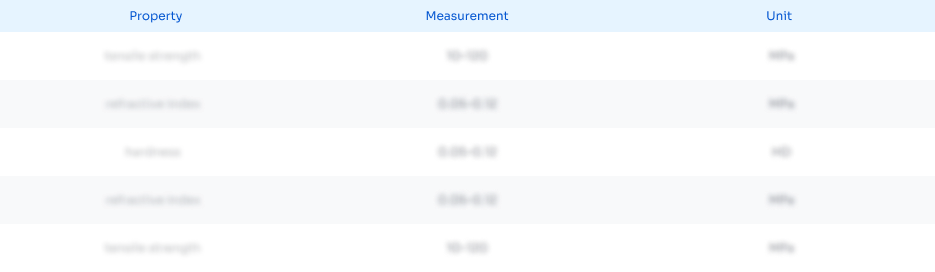
Abstract
Description
Claims
Application Information
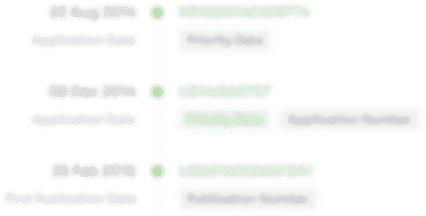
- R&D Engineer
- R&D Manager
- IP Professional
- Industry Leading Data Capabilities
- Powerful AI technology
- Patent DNA Extraction
Browse by: Latest US Patents, China's latest patents, Technical Efficacy Thesaurus, Application Domain, Technology Topic, Popular Technical Reports.
© 2024 PatSnap. All rights reserved.Legal|Privacy policy|Modern Slavery Act Transparency Statement|Sitemap|About US| Contact US: help@patsnap.com