Bearing fault diagnosis method based on improved ant lion algorithm and support vector machine
A technology of support vector machine and fault diagnosis, which is applied in the direction of mechanical bearing testing, computer parts, mechanical parts testing, etc., can solve problems such as increasing falling into local extremum, less research on bearing fault diagnosis, and small search and optimization solution range
- Summary
- Abstract
- Description
- Claims
- Application Information
AI Technical Summary
Problems solved by technology
Method used
Image
Examples
Embodiment
[0166] In order to verify the diagnostic performance of the EALO-SVM algorithm proposed in the present invention, a typical bearing fault vibration test and diagnostic test is carried out. The bearing diagnostic process is as follows figure 2 shown. The test was carried out on a mechanical failure comprehensive simulation test bench of SpectraQuest Company in the United States. The whole device is driven by a three-phase motor, and the output speed of the motor is precisely controlled by PLC mode. The test bench can be disassembled and assembled freely, and is equipped with several typical failure components. In order to better reflect the interference of noise on the vibration signal of the faulty bearing, the faulty bearing was installed on the bearing seat near the motor end during the experiment, and the normal bearing of the same model is on the right. The high-speed multi-channel data acquisition instrument of Austria DEWETRON company is used for data acquisition. A ...
PUM
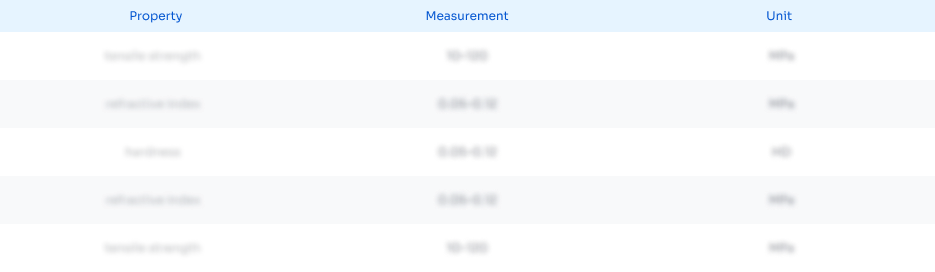
Abstract
Description
Claims
Application Information
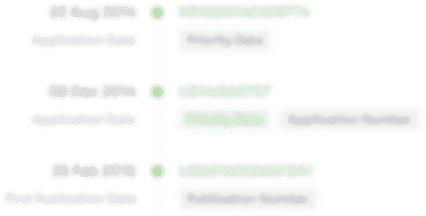
- R&D Engineer
- R&D Manager
- IP Professional
- Industry Leading Data Capabilities
- Powerful AI technology
- Patent DNA Extraction
Browse by: Latest US Patents, China's latest patents, Technical Efficacy Thesaurus, Application Domain, Technology Topic, Popular Technical Reports.
© 2024 PatSnap. All rights reserved.Legal|Privacy policy|Modern Slavery Act Transparency Statement|Sitemap|About US| Contact US: help@patsnap.com