Convolutional neural network-based wind driven generator blade crack detection method
A technology for cracks in wind turbines and blades, applied in the field of computer vision, can solve the problems of high human resource consumption, low efficiency, and heavy detection workload
- Summary
- Abstract
- Description
- Claims
- Application Information
AI Technical Summary
Problems solved by technology
Method used
Image
Examples
Embodiment Construction
[0054] The present invention will be described in further detail below in conjunction with specific examples, but the scope of the present invention is not limited.
[0055] like figure 1 As shown, a method for crack detection of wind turbine blades based on convolutional neural network includes the following steps:
[0056] (1) Collect image samples of wind turbine blades and build training samples for the deep learning model of wind turbine blade images. The specific operation is: manually collect images of wind turbine blades. The image samples of wind turbine blades include intact blades, slightly cracked blades and severely cracked blades, and the variance of the number of samples should not be too large, and the collected sample images should be clear and easy to identify; then all the collected wind turbine blade images are adjusted to the same size ( 784×784×3), use the annotation tool to frame the bounding box of each leaf in the image, and mark the position coordina...
PUM
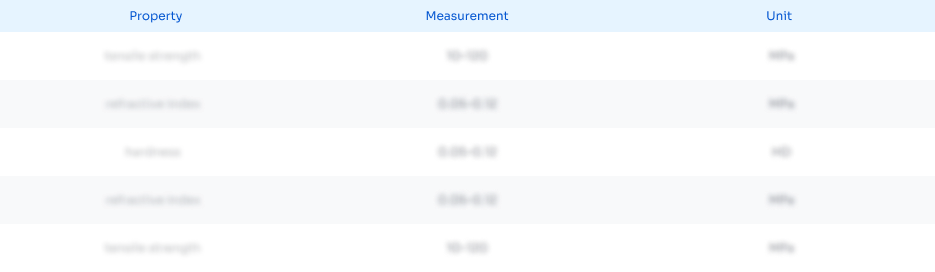
Abstract
Description
Claims
Application Information
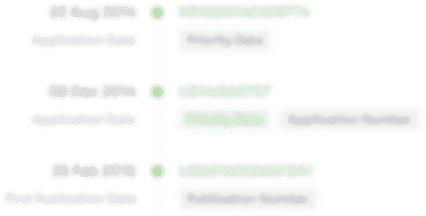
- Generate Ideas
- Intellectual Property
- Life Sciences
- Materials
- Tech Scout
- Unparalleled Data Quality
- Higher Quality Content
- 60% Fewer Hallucinations
Browse by: Latest US Patents, China's latest patents, Technical Efficacy Thesaurus, Application Domain, Technology Topic, Popular Technical Reports.
© 2025 PatSnap. All rights reserved.Legal|Privacy policy|Modern Slavery Act Transparency Statement|Sitemap|About US| Contact US: help@patsnap.com