Brain function network classification method based on variational auto-encoder
A brain function network and autoencoder technology, applied in the field of medical image analysis, can solve the problems of ignoring topological structure relationships, limited data modeling capabilities, and insufficient information in input feature vectors, achieving good distribution characteristics and improving generalization. Ability to achieve the effect of dimensionality reduction
- Summary
- Abstract
- Description
- Claims
- Application Information
AI Technical Summary
Problems solved by technology
Method used
Image
Examples
Embodiment Construction
[0047] A brain function network classification method based on a variational autoencoder of the present invention will be described in detail below in conjunction with the accompanying drawings. Classification of functional brain networks in autistic and normal individuals.
[0048] Such as figure 1 Shown, the present invention comprises the following steps:
[0049] Step 1. Collect enough brain T1-weighted structural images (T1WeightedMRI) and rest state functional MRI (rs-fMRI) images of normal people and autistic patients. In this example, a total of 316 cases were collected. According to the MRI data of the test subjects, 143 of them were diagnosed as autistic, and the remaining 175 were normal.
[0050] Step 2: Preprocessing the collected structural MRI images and functional MRI images, extracting the brain from T1-weighted structural images, cortical reconstruction, head motion estimation and correction, slice time correction, intra-individual registration, global norm...
PUM
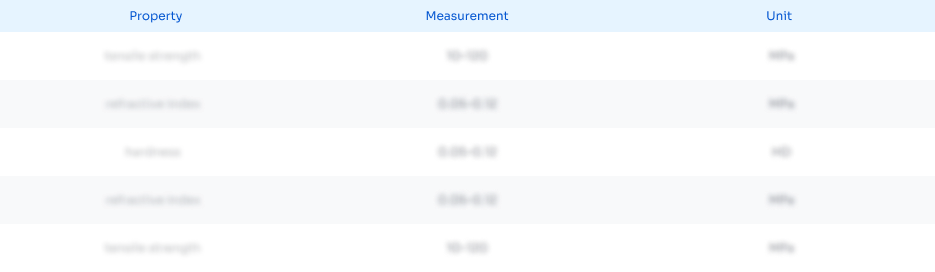
Abstract
Description
Claims
Application Information
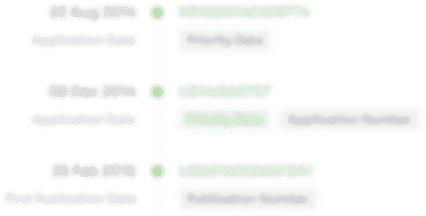
- R&D Engineer
- R&D Manager
- IP Professional
- Industry Leading Data Capabilities
- Powerful AI technology
- Patent DNA Extraction
Browse by: Latest US Patents, China's latest patents, Technical Efficacy Thesaurus, Application Domain, Technology Topic, Popular Technical Reports.
© 2024 PatSnap. All rights reserved.Legal|Privacy policy|Modern Slavery Act Transparency Statement|Sitemap|About US| Contact US: help@patsnap.com