Steel rail scale damage detection method based on deep learning
A technology of deep learning and detection methods, applied in neural learning methods, image data processing, instruments, etc., can solve problems such as asymmetry, consumption, large labor costs and time costs, and achieve high accuracy, good learning ability, high Effect of Detection Efficiency and Accuracy
- Summary
- Abstract
- Description
- Claims
- Application Information
AI Technical Summary
Problems solved by technology
Method used
Image
Examples
Embodiment Construction
[0057] Main implementation steps of the present invention are as follows:
[0058] 1. Division of data sets
[0059] Divide the data set into training set, test set and verification set according to the ratio of 7:2:1. The training set is mainly used for model fitting to data samples and to find the regularity between samples. The test set is mainly used in the training process to determine the parameters of the network structure or control the complexity of the model. The test set is used to evaluate the entire neural learning situation, that is, to verify the accuracy of the method for the detection of fish scale damage at various levels.
[0060] 2. Network structure setting and forward propagation
[0061] In the present invention, the residual neural network is used for training deep learning training. The residual neural network is mainly composed of convolutional layers, pooling layers and fully connected layers. Among them, the convolutional layer of the residual ...
PUM
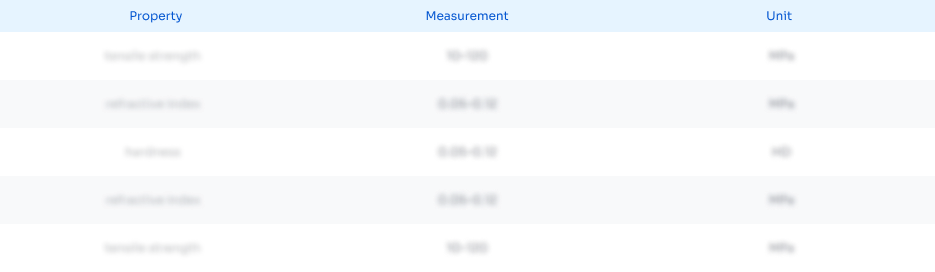
Abstract
Description
Claims
Application Information
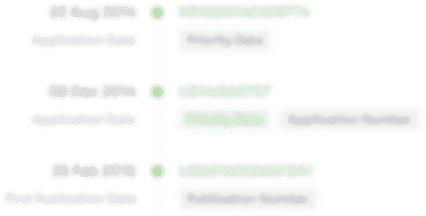
- R&D Engineer
- R&D Manager
- IP Professional
- Industry Leading Data Capabilities
- Powerful AI technology
- Patent DNA Extraction
Browse by: Latest US Patents, China's latest patents, Technical Efficacy Thesaurus, Application Domain, Technology Topic, Popular Technical Reports.
© 2024 PatSnap. All rights reserved.Legal|Privacy policy|Modern Slavery Act Transparency Statement|Sitemap|About US| Contact US: help@patsnap.com