Method for intelligently coloring microstructure photo shot by electron microscope and CNN coloring learner
An electron microscope and microstructure technology, applied in instruments, image data processing, computing, etc., can solve the problems of a large number of user parameter input, spend a lot of time and energy, consume knowledge and time, and achieve less training and testing, and faster coloring efficiency. , train and test fast results
- Summary
- Abstract
- Description
- Claims
- Application Information
AI Technical Summary
Problems solved by technology
Method used
Image
Examples
Embodiment 1
[0043] join figure 1 with 3 , this embodiment provides a method for intelligently coloring end-to-end microstructure photos taken by an electron microscope, including the following steps:
[0044] Step ①: First create a data set, which is a collection of about 1,000 color SEM images. The pictures come from daily life, and the images have different shapes. The shader learns the above data set, and the learning method is based on Google's machine learning Technology, you can refer to the existing literature GoogleResearch.TensorFlow:Large-scale machine learning on heterogeneous systems.Google Res.(2015).doi:10.1207 / s15326985ep4001; Lecun,Y.,Bengio,Y.&Hinton,G.Deep learning.Nature( 2015).doi:10.1038 / nature14539;
[0045] Step ②: Convert the gray-scale photo of the chromosome to be colored taken by the electron microscope into the LAB color space as the input image, L is the brightness, A represents the range from red to green, B represents the range from yellow to blue, and the...
Embodiment 2
[0048] Such as figure 2 with 4 As shown, this embodiment demonstrates a method for intelligently coloring pixel-to-pixel microstructure photos taken by an electron microscope. The method is based on the CNN multi-convolution layer framework and has multiple channels. The three grayscale photos in this embodiment are electron microscope scanning photos of viruses, red blood cells, and neurons from left to right.
[0049] Manually select a natural color photo in real life as a reference picture for color style transfer, decode the picture and convert its format from RBG to LAB, and obtain the L gray channel Y L and AB color channel Y AB , so as to facilitate the later input to the encoder under the CNN framework of the convolutional neural network. Encoder pair input Y L The color image for coloring output prediction is the predicted channel Y AB ’, the standard Y AB and Y AB ’ The mean square error (MSE) loss generated is passed back to train the convolutional neural ne...
Embodiment 3
[0053] see Figure 5 , many SEM grayscale images have more repeated similar units. This embodiment uses the grayscale of uniform dense objects in the same reference color image for two different SEM grayscale images of polystyrene nanospheres. The image undergoes end-to-end color style transfer and coloring, and the final output results are in line with the laws of human vision.
PUM
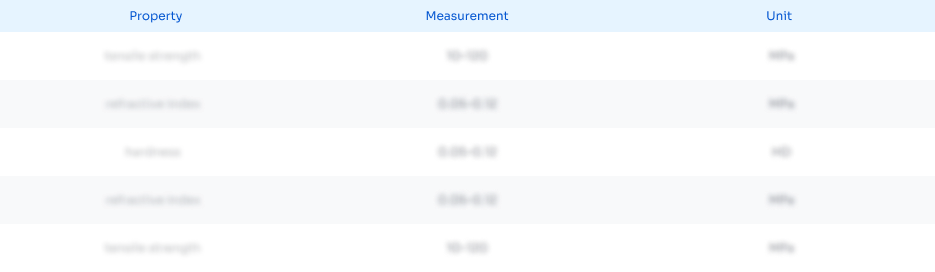
Abstract
Description
Claims
Application Information
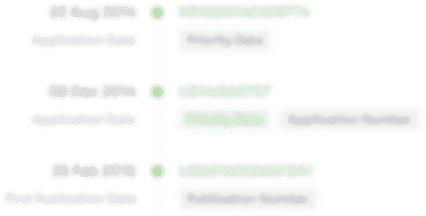
- R&D
- Intellectual Property
- Life Sciences
- Materials
- Tech Scout
- Unparalleled Data Quality
- Higher Quality Content
- 60% Fewer Hallucinations
Browse by: Latest US Patents, China's latest patents, Technical Efficacy Thesaurus, Application Domain, Technology Topic, Popular Technical Reports.
© 2025 PatSnap. All rights reserved.Legal|Privacy policy|Modern Slavery Act Transparency Statement|Sitemap|About US| Contact US: help@patsnap.com