Big data cluster self-adaptive resource scheduling method based on cloud platform
A resource scheduling and big data technology, applied in the field of cloud computing, can solve problems such as difficult to meet the requirements of rapid response in complex and changeable environments, and achieve the effect of improving resource utilization, reducing total cost, and ensuring resource utilization
- Summary
- Abstract
- Description
- Claims
- Application Information
AI Technical Summary
Problems solved by technology
Method used
Image
Examples
Embodiment 1
[0055] The present invention configures big data cluster resources for different types of tasks under the cloud platform, such as Figure 9 As shown, when a user needs to apply for resources to run a task, first, classify and analyze the big data analysis task, and use a three-layer neural network classifier to classify the task into a pre-marked type; then, in a small number of samples In the preliminary stage of cluster configuration, the Bayesian optimization method is used to find the configuration that minimizes the cost of resources requested by the user, and returns it to the user; after that, an online optimization module is added based on the idea of data-driven, and the real Iterative dynamic optimization of time to solve problems such as inaccurate classification that may exist in the previous stage (the first three stages are workflows with no time limit, such as figure 2 shown); Finally, for tasks with time constraints, use NNLS (non-negative least squares) to ...
Embodiment 2
[0134] 1) Big data analysis task classification analysis experiment
PUM
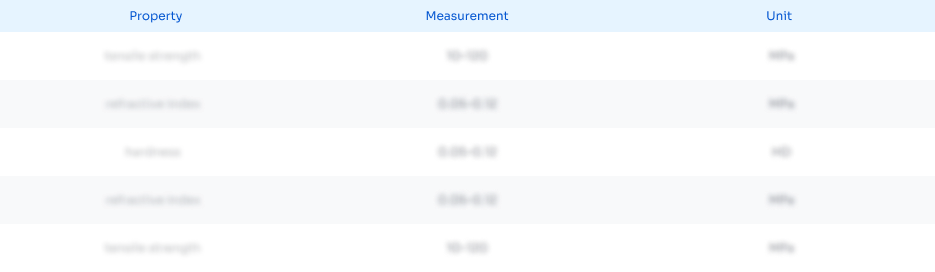
Abstract
Description
Claims
Application Information
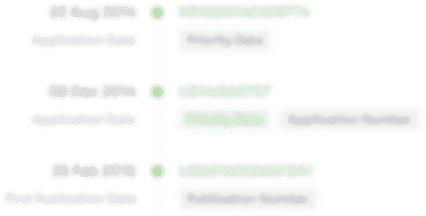
- R&D
- Intellectual Property
- Life Sciences
- Materials
- Tech Scout
- Unparalleled Data Quality
- Higher Quality Content
- 60% Fewer Hallucinations
Browse by: Latest US Patents, China's latest patents, Technical Efficacy Thesaurus, Application Domain, Technology Topic, Popular Technical Reports.
© 2025 PatSnap. All rights reserved.Legal|Privacy policy|Modern Slavery Act Transparency Statement|Sitemap|About US| Contact US: help@patsnap.com