Unsupervised monocular depth estimation method based on generative adversarial network
A depth estimation and unsupervised technology, applied in the field of robot vision, can solve the problems of high sensor cost and inaccurate camera pose estimation, and achieve the effect of improving accuracy and image generation quality
- Summary
- Abstract
- Description
- Claims
- Application Information
AI Technical Summary
Problems solved by technology
Method used
Image
Examples
Embodiment Construction
[0052] Such as figure 1 As shown, an unsupervised monocular depth estimation method based on generating confrontation network of the present invention includes the following steps:
[0053] Step 1: Acquire the left and right image pairs with strict time synchronization through the binocular camera, establish a binocular color image dataset, and correct the binocular color image;
[0054] Step 2: Establish an unsupervised generative confrontation network model, input the corrected binocular color image into the network, and perform training and iterative regression on the network model;
[0055] Step 3: Input the monocular color image into the trained network model to generate the corresponding disparity map;
[0056] The unsupervised generation confrontation network model established by the present invention includes a generator and a discriminator, the generator uses a ResNet50 network with a residual mechanism, and the discriminator uses a VGG-16 network. The generator inc...
PUM
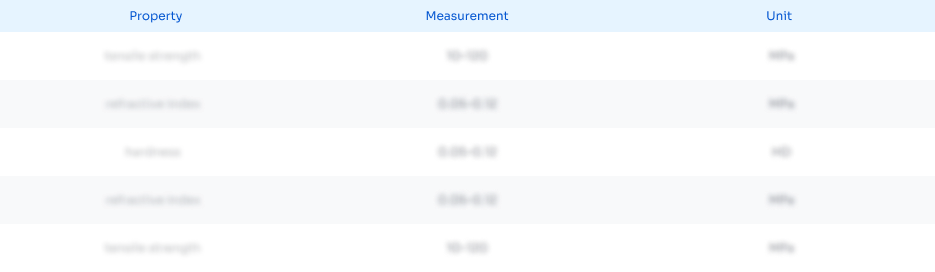
Abstract
Description
Claims
Application Information
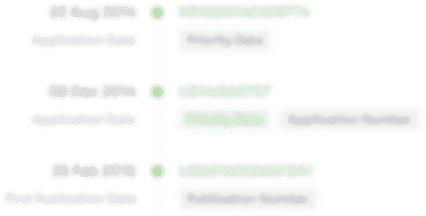
- R&D Engineer
- R&D Manager
- IP Professional
- Industry Leading Data Capabilities
- Powerful AI technology
- Patent DNA Extraction
Browse by: Latest US Patents, China's latest patents, Technical Efficacy Thesaurus, Application Domain, Technology Topic, Popular Technical Reports.
© 2024 PatSnap. All rights reserved.Legal|Privacy policy|Modern Slavery Act Transparency Statement|Sitemap|About US| Contact US: help@patsnap.com