Winding fault locating method for power transformer based on fusion of deep convolutional neural network and visual identification
A power transformer and neural network technology, applied in the field of power transformer fault diagnosis and location, can solve problems such as limiting the applicability of fault diagnosis methods, and achieve the effects of improving positioning accuracy, facilitating fault diagnosis, and various processing methods
- Summary
- Abstract
- Description
- Claims
- Application Information
AI Technical Summary
Problems solved by technology
Method used
Image
Examples
Embodiment Construction
[0048] Next, the present invention will be further described in conjunction with the drawings and embodiments.
[0049] The invention can effectively realize transformer winding fault identification and positioning; overcome the shortcomings of traditional sweep frequency response analysis and judgment faults that rely too much on expert experience and have no unified standards; intelligent classification using convolutional neural networks can simplify application difficulty and improve reliability and applicability and positioning accuracy. The invention proposes a power vision method that converts the detection waveform into an image and uses a convolutional neural network for fault diagnosis, which can intuitively reflect fault characteristics, make the data type suitable for the input requirements of the convolutional neural network, and facilitate observation and verification of the output results.
[0050] Such as figure 1 As shown, the power transformer winding fault ...
PUM
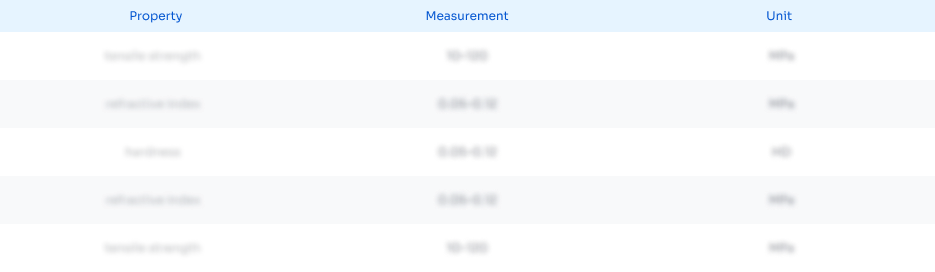
Abstract
Description
Claims
Application Information
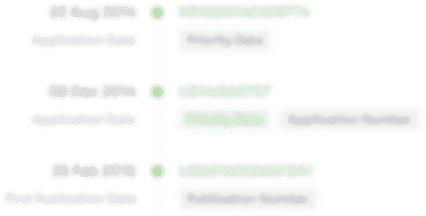
- R&D Engineer
- R&D Manager
- IP Professional
- Industry Leading Data Capabilities
- Powerful AI technology
- Patent DNA Extraction
Browse by: Latest US Patents, China's latest patents, Technical Efficacy Thesaurus, Application Domain, Technology Topic, Popular Technical Reports.
© 2024 PatSnap. All rights reserved.Legal|Privacy policy|Modern Slavery Act Transparency Statement|Sitemap|About US| Contact US: help@patsnap.com