Micro-Doppler radar human body action classification method of convolutional neural network
A technology of convolutional neural network and human action, which is applied in the direction of radio wave reflection/reradiation, instrumentation, computing, etc., and can solve the problems that the end-to-end learning advantages of the neural network cannot be fully utilized.
- Summary
- Abstract
- Description
- Claims
- Application Information
AI Technical Summary
Problems solved by technology
Method used
Image
Examples
specific Embodiment
[0062] Use Infineon's Sense2GoL Doppler radar to acquire micro-Doppler signals for human sleep movements and human daily movements. The radar chip used in Sense2GoL is BGT24LTR11, which is a silicon-germanium MMIC (single-chip microwave integrated circuit) for signal transmission and reception, and the operating frequency is from 24.05GHz to 24.25GHz. The Sense2GoL Doppler Radar has a maximum power of 10mW and it combines a receiver and a transmitter. RadarNet is trained using a server with 32G memory and NVIDIA GTX1080Ti graphics card.
[0063] Collect radar data for both experiments with a sampling frequency of 2 kHz for 3 s. The size of the STFT time window was set to 25.5 ms, and the overlapping time step was set to 6 ms. Correspondingly, the size of the one-dimensional convolution kernel L is set to 51 and the stride size is 39 in RadarNet. The number of kernels of a one-dimensional convolutional layer is set to 150, which corresponds to a STFT of 150 points. The enti...
Embodiment 1
[0064] Embodiment 1 Human body sleep action classification:
[0065] Doppler radar was used to collect data on three types of human sleep activity: (a) turning over, (b) hand movements and (c) head movements. The data set is derived from four individuals. Data collection scenarios such as Figure 8 shown. The radar is placed on one side of the bed, at a distance of about 20 cm from the bed, at the same level as the bed. For a more sensitive signal, the radar detection range is roughly located on the chest. The collection time for each action is 3 seconds. The distribution of action data is shown in Table 1. The original signal waveforms of three human sleep movements are as follows: Figure 9 shown.
[0066] Table 1 The categories and groups of human sleep movements
[0067]
[0068] In this experiment, STFTNet is compared with the described RadarNet using 5-fold cross-validation. The total training duration is 5,814 seconds for RadarNet and 4,237 seconds for STFTN...
Embodiment 2
[0074] Embodiment 2 Human body's daily action classification:
[0075] In order to verify the flexibility and robustness of the RadarNet of the present invention, another more complex experiment can be performed to classify the daily actions of the human body. Doppler radar was used to collect data on seven types of human daily activities: (a) running, (b) walking, (c) walking with a gun, (d) crawling, (e) walking boxing, (f) standing boxing, ( g) sit still. These types of actions are the same as those used in the study by Kim et al. [1]. This dataset collects data from 4 individuals. The postures of these seven movements are as follows: Figure 11 shown. The radar is placed at a height of 1.2 meters above the ground. Actions (a), (b), (c), (d) and (e) were collected by the experimenter moving forward from a distance of 5 m to the radar. The remaining two activities were collected at 0.5 meters from the radar. The collection time for each activity of (a), (b), (c), (d) ...
PUM
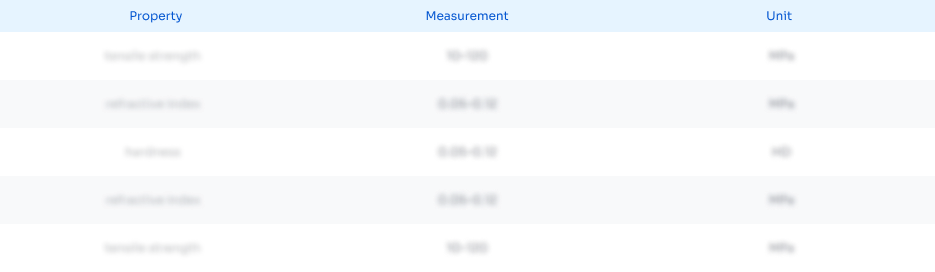
Abstract
Description
Claims
Application Information
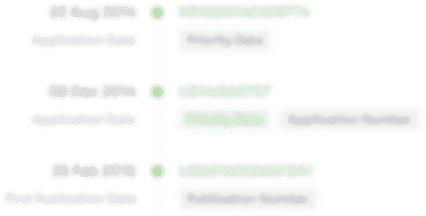
- R&D
- Intellectual Property
- Life Sciences
- Materials
- Tech Scout
- Unparalleled Data Quality
- Higher Quality Content
- 60% Fewer Hallucinations
Browse by: Latest US Patents, China's latest patents, Technical Efficacy Thesaurus, Application Domain, Technology Topic, Popular Technical Reports.
© 2025 PatSnap. All rights reserved.Legal|Privacy policy|Modern Slavery Act Transparency Statement|Sitemap|About US| Contact US: help@patsnap.com