LSTM-RNN-based combined cooling heating and power system load prediction method and system
A technology of combined cooling, heating and power supply and system load, applied in forecasting, neural learning methods, data processing applications, etc., can solve the problems of rarely considering the coupling relationship and inapplicability, and achieve the effect of improving prediction accuracy and accuracy
- Summary
- Abstract
- Description
- Claims
- Application Information
AI Technical Summary
Problems solved by technology
Method used
Image
Examples
Embodiment 1
[0052] This embodiment discloses a LSTM-RNN-based load forecasting method for combined cooling, heating and power generation systems based on long-short-term memory recurrent neural networks, such as figure 2 shown, including the following steps:
[0053] Step 1: Calculate the correlation of historical data and determine the input and output of the model.
[0054]Specifically, in the step 1, the autocorrelation coefficient, partial correlation coefficient and Pearson correlation coefficient are respectively used to analyze the correlation between historical data, so as to select appropriate data as input and output variables.
[0055] The autocorrelation coefficient is used to measure the degree of correlation between the same event in two different periods. Specifically, with a certain moment as a reference, the autocorrelation coefficient of each moment in the moment and a certain period of time before it is calculated respectively. Its formula can be expressed as:
[005...
Embodiment 2
[0100] The purpose of this embodiment is to provide a load forecasting system for a combined cooling, heating and power system.
[0101] In order to achieve the above purpose, this embodiment provides a load forecasting system for a combined cooling, heating and power system, including:
[0102] The data acquisition module receives the historical data of heating load, cooling load and electric load;
[0103] The correlation analysis module determines the time period of the input data according to the correlation between a certain moment of historical data and multiple moments before it; determines the input and output according to the correlation coefficient between the historical data of heating load, cooling load and electric load data;
[0104] The model training module inputs the training set into the long-short-term memory recurrent neural network, and trains the load prediction network model;
[0105] A load forecasting module performs load forecasting based on the loa...
Embodiment 3
[0107]The purpose of this embodiment is to provide an electronic device.
[0108] An electronic device, comprising a memory, a processor, and a computer program stored on the memory and operable on the processor, when the processor executes the program, the following steps are implemented, including:
[0109] Receive historical data of heating load, cooling load and electrical load;
[0110] Determine the time period for input data based on the correlation between a certain moment of historical data and multiple moments before it;
[0111] Determine the input and output data according to the correlation coefficient between the historical data of heating load, cooling load and electric load;
[0112] Input the training set into the long short-term memory recurrent neural network to train the load prediction network model;
[0113] Load forecasting is performed based on the load forecasting network model.
PUM
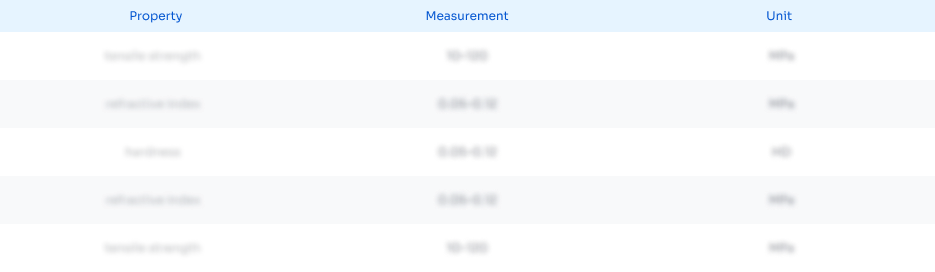
Abstract
Description
Claims
Application Information
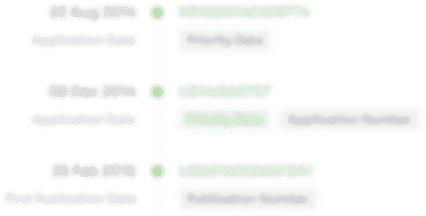
- R&D
- Intellectual Property
- Life Sciences
- Materials
- Tech Scout
- Unparalleled Data Quality
- Higher Quality Content
- 60% Fewer Hallucinations
Browse by: Latest US Patents, China's latest patents, Technical Efficacy Thesaurus, Application Domain, Technology Topic, Popular Technical Reports.
© 2025 PatSnap. All rights reserved.Legal|Privacy policy|Modern Slavery Act Transparency Statement|Sitemap|About US| Contact US: help@patsnap.com