Multi-feature cyclic convolution saliency target detection method based on attention mechanism
A cyclic convolution and target detection technology, applied in image data processing, image enhancement, instruments, etc., can solve problems such as insufficient feature utilization, blurred target contour, background error detection, etc., to enhance spatial resolution and enhance feature representation. effect of ability
- Summary
- Abstract
- Description
- Claims
- Application Information
AI Technical Summary
Problems solved by technology
Method used
Image
Examples
Embodiment Construction
[0035] The present invention will be further described below in conjunction with the accompanying drawings.
[0036] A multi-feature circular convolution salient target detection method based on the attention mechanism provided by the present invention, the overall structure of the method is as follows figure 1 shown. In this method, U-Net is selected as the backbone network, and an inter-pixel-inter-channel double attention module is designed to enhance the utilization efficiency of features and reduce noise and background pixel interference; a circular convolution module is designed to refine the edge contours of significant regions through cyclic iterations; Multi-stage constraints on prediction results using a bypass output strategy. The dual-attention module proposed by the present invention considers attention from two perspectives of channel and space, calibrates the response weight between channels, highlights the pixel response strength of the foreground area, enhanc...
PUM
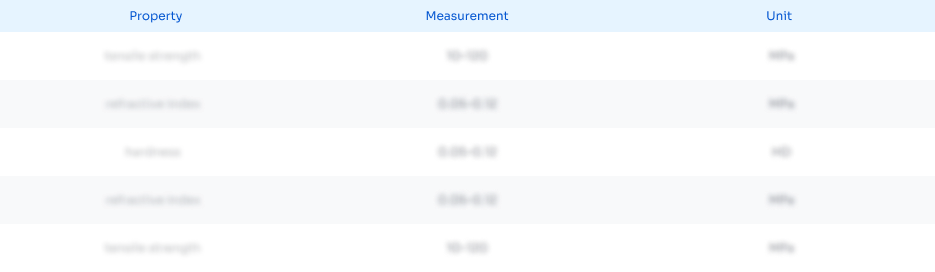
Abstract
Description
Claims
Application Information
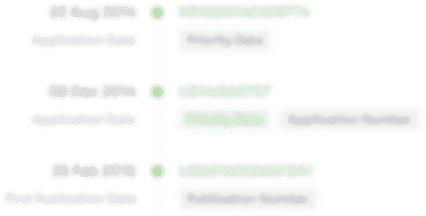
- R&D Engineer
- R&D Manager
- IP Professional
- Industry Leading Data Capabilities
- Powerful AI technology
- Patent DNA Extraction
Browse by: Latest US Patents, China's latest patents, Technical Efficacy Thesaurus, Application Domain, Technology Topic, Popular Technical Reports.
© 2024 PatSnap. All rights reserved.Legal|Privacy policy|Modern Slavery Act Transparency Statement|Sitemap|About US| Contact US: help@patsnap.com