Robot multi-joint self-adaptation compensation method based on perceptron model and stabilizing device
An adaptive compensation and robot technology, applied in the control field, can solve the problems of fixed parameters, limited compensation range of a single-joint stabilizer, and inability to deal with interference, so as to eliminate the center of mass tracking error and body posture error, and avoid the parameter calculation and tuning process. , Improve the effect of network convergence speed
- Summary
- Abstract
- Description
- Claims
- Application Information
AI Technical Summary
Problems solved by technology
Method used
Image
Examples
Embodiment 1
[0050] Such as figure 1 As shown, this embodiment is a multi-joint adaptive compensation method for a robot based on a perceptron model, and the method includes the following steps:
[0051] S1: Obtain error data based on the robot inertial sensor and gait generator;
[0052] The error data includes attitude angle error, attitude angular velocity error, robot center of mass tracking error and first order difference of center of mass error. Attitude angle e θ and angular velocity error It can be directly measured by the robot's inertial sensors (gyroscope and accelerometer); the gait generator plans the trajectory of the center of mass, and makes a difference from the actual measured center of mass to obtain the center of mass error e CoM , and then make a first-order difference to get the centroid error differential term
[0053] S2: Input the error data into the pre-established perceptron model, the perceptron model updates the network weight parameters based on the as...
Embodiment 2
[0076] This embodiment is a robot multi-joint adaptive compensation stabilizer based on the perceptron model. The control objects of this embodiment are the trajectory of the robot's center of mass and the posture of the upper body; in order to improve robustness, resist large disturbances, and reduce the load on a single joint of the robot , the actuator is no longer the hip joint or the ankle joint, but the compensation is distributed to all joints of the leg (ankle joint, knee joint, hip joint). Considering that the traditional stabilizer often adopts PD control, and in order to eliminate the center of mass error and attitude error during the walking process of the robot at the same time, the input of the stabilizer in this embodiment is determined as body attitude angle error, angular velocity error, center of mass tracking error and first order difference of center of mass error ; The output is the compensation amount of each joint of the robot leg. In this embodiment, a ...
specific Embodiment approach
[0107] The experimental platform of this example is the Nao humanoid robot of SoftBank Company. The MPC gait planner is used to generate the basic walking gait of the robot. On this basis, the multi-joint self-adaptive compensation stabilizer of the present invention is used to compensate the leg joints of the robot. Eliminate the tracking error of the center of mass trajectory during walking and adjust the posture of the upper body of the robot to improve the stability of walking and adaptability to the environment.
[0108] 1. Parameter initialization
[0109] Such as Figure 4 As shown, the network hyperparameters in this stabilizer include output gain (K) and weight learning rate η. Figure 4 are the structural parameters of the Nao humanoid robot used in this example, wherein Nao's torso is 85mm away from the hip joint (hip), 185mm away from the knee joint (knee), and 287mm away from the ankle joint (ankle). The sensitivity of the center of mass is roughly inversely pro...
PUM
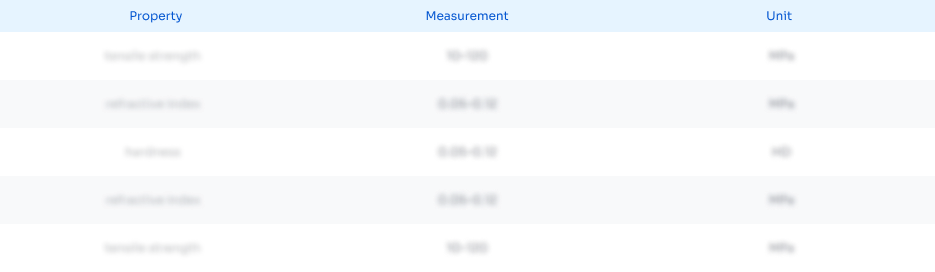
Abstract
Description
Claims
Application Information
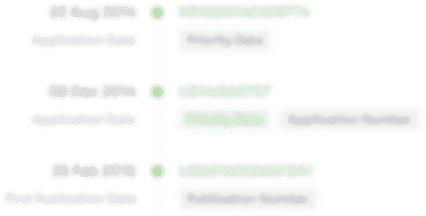
- R&D
- Intellectual Property
- Life Sciences
- Materials
- Tech Scout
- Unparalleled Data Quality
- Higher Quality Content
- 60% Fewer Hallucinations
Browse by: Latest US Patents, China's latest patents, Technical Efficacy Thesaurus, Application Domain, Technology Topic, Popular Technical Reports.
© 2025 PatSnap. All rights reserved.Legal|Privacy policy|Modern Slavery Act Transparency Statement|Sitemap|About US| Contact US: help@patsnap.com