Distributed reinforcement learning stable topology generation method based on adaptive boundary
A technology of reinforcement learning and topology generation, applied in the field of communication, can solve problems such as long execution time, no consideration of the comprehensive impact of links, network communication congestion, etc., to improve network communication quality, enhance link connection time, and avoid network overhead. Effect
- Summary
- Abstract
- Description
- Claims
- Application Information
AI Technical Summary
Problems solved by technology
Method used
Image
Examples
Embodiment 1
[0035] The mobile ad hoc network plays an important role in the communication network without infrastructure. The network has no infrastructure support. Each mobile node has both router and host functions, and can form any network topology through wireless connection. Mobile ad hoc networks have broad application prospects in military communications, mobile networks, connecting personal area networks, emergency services and disaster recovery, and wireless sensor networks. Therefore, the mobile ad hoc network has also become one of the current research hotspots. The mobility of nodes in the mobile ad hoc network causes the network topology formed by the entire wireless channel to change at any time. In order to effectively reduce the impact of dynamic topology changes, the existing methods use the mobility of nodes to predict the link connection in the network. The degree of stability and network topology to reduce the impact of dynamic topology changes. However, the existing ...
Embodiment 2
[0055] The distributed reinforcement learning stable topology generation method based on adaptive boundaries is the same as that in Embodiment 1, and the reinforcement learning method described in step 4 of the present invention, the specific implementation process includes the following steps:
[0056] Step 4.1 Determine the overall structure of the reinforcement learning method: In the interval [a,b], each node in the mobile ad hoc network is regarded as an agent agent, and the dynamic changes of MANET can be regarded as a distributed multi-agent collaborative system . For each distributed agent Agent, it is assumed that its environment state set is S, the action set is A, and the reward function is The action selection strategy is π(s i ,a j ).
[0057] The present invention builds a reinforcement learning model in the mobile self-organizing network, regards the network as a multi-agent cooperative system, effectively combines the scene of the mobile self-organizing net...
Embodiment 3
[0079] The distributed reinforcement learning stable topology generation method based on adaptive boundaries is the same as that in Embodiment 1-2, and the adaptive interval boundary update formula in step 6 of the present invention is as follows:
[0080]
[0081] In the formula: a is the upper boundary of the interval; b is the lower boundary of the interval; RSSI is the received signal strength indicator value of the neighbor node; s' is the actual connection variable state of the node and the neighbor node at the next moment; For the prediction of the connection variable state between the node and the neighbor node at the next moment; in the present invention, adaptive_rate is set as the proportional coefficient of the adaptive boundary adjustment, that is, the ratio of the connection state prediction error times and the total number of predictions at the next transmission moment predicted by the current node . like When a0.1, adjust the adaptive boundary a=RSSI; if ...
PUM
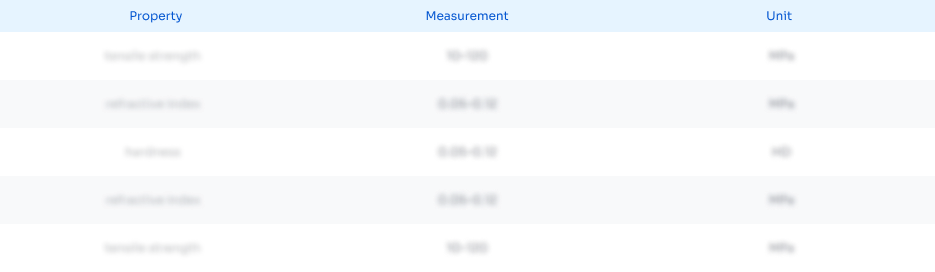
Abstract
Description
Claims
Application Information
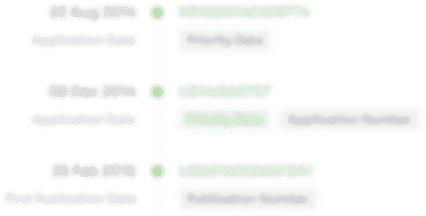
- R&D Engineer
- R&D Manager
- IP Professional
- Industry Leading Data Capabilities
- Powerful AI technology
- Patent DNA Extraction
Browse by: Latest US Patents, China's latest patents, Technical Efficacy Thesaurus, Application Domain, Technology Topic, Popular Technical Reports.
© 2024 PatSnap. All rights reserved.Legal|Privacy policy|Modern Slavery Act Transparency Statement|Sitemap|About US| Contact US: help@patsnap.com