Image deblurring method based on multi-task CNN
A deblurring, multi-tasking technology, applied in image enhancement, image analysis, image data processing, etc., can solve the problems of loss of detailed texture, insufficient generalization ability of network model, and failure to consider image detail information recovery, etc., to achieve enhanced recovery. Effect
- Summary
- Abstract
- Description
- Claims
- Application Information
AI Technical Summary
Problems solved by technology
Method used
Image
Examples
Embodiment Construction
[0013] The present invention will be described in further detail below in conjunction with the accompanying drawings and specific embodiments. It should be understood that the specific embodiments described here are only used to explain the present invention, not to limit the present invention.
[0014] The present invention proposes to increase the convolutional neural network for detail recovery, designs an image detail recovery module to fully extract and protect the detail features of fuzzy images, and proposes to increase the gradient loss to enhance the network's ability to recover details. In the overall structure, based on the network structure of Multi-scale [6], a multi-task end-to-end learning defuzzification CNN network is proposed.
[0015] 1. Basic principles of the algorithm
[0016] At present, most convolutional neural networks do not consider the restoration of image detail information in the deblurring process, resulting in the loss of detailed texture afte...
PUM
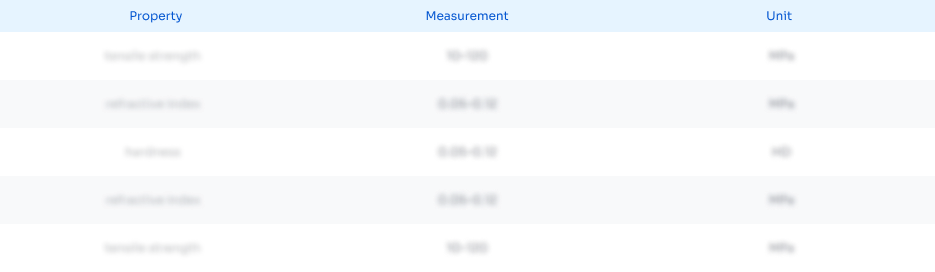
Abstract
Description
Claims
Application Information
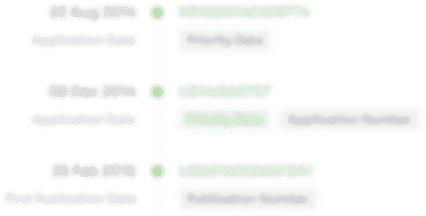
- R&D Engineer
- R&D Manager
- IP Professional
- Industry Leading Data Capabilities
- Powerful AI technology
- Patent DNA Extraction
Browse by: Latest US Patents, China's latest patents, Technical Efficacy Thesaurus, Application Domain, Technology Topic, Popular Technical Reports.
© 2024 PatSnap. All rights reserved.Legal|Privacy policy|Modern Slavery Act Transparency Statement|Sitemap|About US| Contact US: help@patsnap.com