Full convolutional network fabric defect detection method based on attention mechanism
A fully convolutional network and detection method technology, applied in the field of fabric defect detection based on the attention mechanism, can solve the problems of low detection accuracy and slow detection speed, and achieve improved representation ability, improved effectiveness, and good The effect of detection accuracy and adaptability
- Summary
- Abstract
- Description
- Claims
- Application Information
AI Technical Summary
Problems solved by technology
Method used
Image
Examples
specific example
[0061] In the embodiment, randomly select several types of common defect images from a database containing 2000 fabric images, such as image 3 (a) ~ (d), where image 3 (a) is a hole, image 3 (b) is a foreign body, image 3 (c) is a stain, image 3(d) is oil stain, and the image size is selected as 512pixel×512pixel. During training and testing, the learning rate is set to 1E-6, the momentum parameter is set to 0.9, the weight decay is set to 0.0005, and the loss weight for each stage output is chosen to be 1.0. The fusion weights in the feature fusion module are all initialized to 0.2 during the training phase. For specific examples, see Figure 4 ~ Figure 7 .
[0062] Figure 4 (a)~(d) are the truth maps labeled pixel by pixel. Figure 5 (a)~(d) are the literature [1]-[Hou Q, ChengM M, Hu X, et al.Deeply Supervised Salient Object Detection with ShortConnections[J].IEEE Transactions on Pattern Analysis and Machine Intelligence,2018:1- 1.] The saliency map generated...
PUM
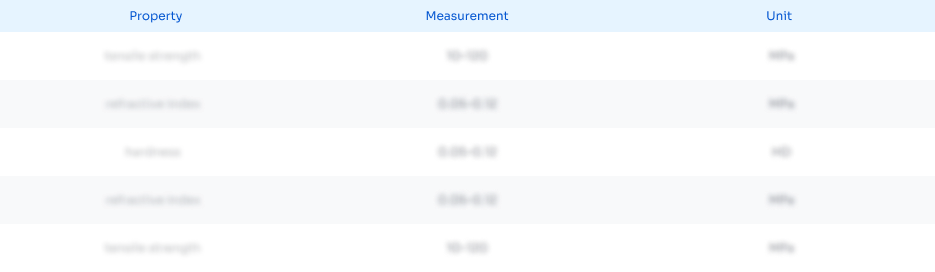
Abstract
Description
Claims
Application Information
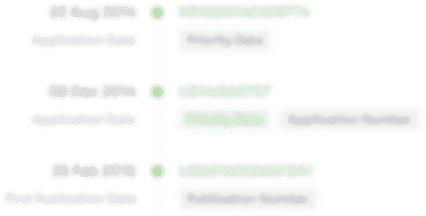
- R&D
- Intellectual Property
- Life Sciences
- Materials
- Tech Scout
- Unparalleled Data Quality
- Higher Quality Content
- 60% Fewer Hallucinations
Browse by: Latest US Patents, China's latest patents, Technical Efficacy Thesaurus, Application Domain, Technology Topic, Popular Technical Reports.
© 2025 PatSnap. All rights reserved.Legal|Privacy policy|Modern Slavery Act Transparency Statement|Sitemap|About US| Contact US: help@patsnap.com