Pattern Recognition Method of Transformer Multi-source Partial Discharge Based on Parallel Eigen Domain
A partial discharge and pattern recognition technology, applied in character and pattern recognition, neural learning methods, instruments, etc., can solve the problems of inaccurate signal feature quantity and poor signal separation effect, achieve strong sparse ability, improve generalization ability, The effect of performance improvement
- Summary
- Abstract
- Description
- Claims
- Application Information
AI Technical Summary
Problems solved by technology
Method used
Image
Examples
Embodiment Construction
[0070] figure 1 It is a structural diagram of the autoencoder of the present invention; it can be seen that the autoencoder is an unsupervised neural network with only one hidden layer. The neural network is composed of an encoder network and a decoder network. The encoder network is used to construct the feature space and has excellent feature extraction capabilities, while the decoder network can reconstruct the input data from the feature space. The encoder network maps the input layer to the hidden layer through the activation function, where the activation value of each neuron in the input layer corresponds to each value of the input data, and the activation value of the hidden layer neuron is the weight matrix, bias matrix and Non-linear mapping values between input data.
[0071] figure 2 It is a schematic diagram of the parallel feature domain of the present invention; it can be seen that the parallel feature domain is composed of two stacked encoders, one of whic...
PUM
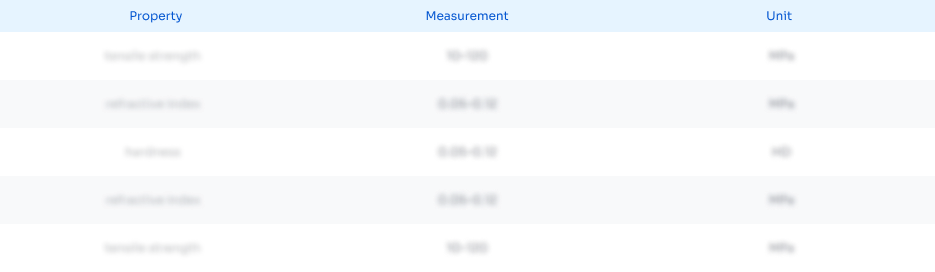
Abstract
Description
Claims
Application Information
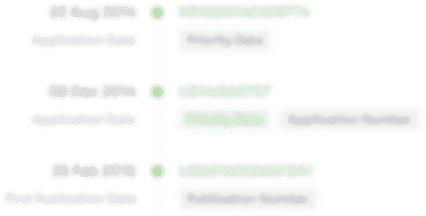
- R&D Engineer
- R&D Manager
- IP Professional
- Industry Leading Data Capabilities
- Powerful AI technology
- Patent DNA Extraction
Browse by: Latest US Patents, China's latest patents, Technical Efficacy Thesaurus, Application Domain, Technology Topic.
© 2024 PatSnap. All rights reserved.Legal|Privacy policy|Modern Slavery Act Transparency Statement|Sitemap