Graph model based on multi-view dictionary learning
A dictionary learning and multi-view technology, applied in the field of graphical models based on multi-view dictionary learning, can solve the problems of multi-view technology application, high computing resource consumption, low information utilization rate, etc., and achieve high information utilization rate and data discrimination Strong and generalizable effect
- Summary
- Abstract
- Description
- Claims
- Application Information
AI Technical Summary
Problems solved by technology
Method used
Image
Examples
Embodiment Construction
[0022] The present invention will be described in further detail below with reference to the embodiments and the accompanying drawings, but the embodiments of the present invention are not limited thereto.
[0023] The present invention is a graph model based on multi-view dictionary learning, which uses Principal Component Analysis (Principal Component Analysis) and Linear Discrimination Analysis (Linear Discrimination Analysis) to perform data dimension reduction and preprocessing on the original graph data to remove redundant features in the data. While retaining the high discriminativeness of the data; then use the multi-view dictionary learning method to learn the essential features contained in the data, and train to obtain a synthetic dictionary (SynthesisDictionary), an analysis dictionary (Analysis Dictionary), and sparse coding corresponding to the sample (SparseCode) and an SVM linear classifier; then the sparse coding of the sample is input into the SVM classifier, ...
PUM
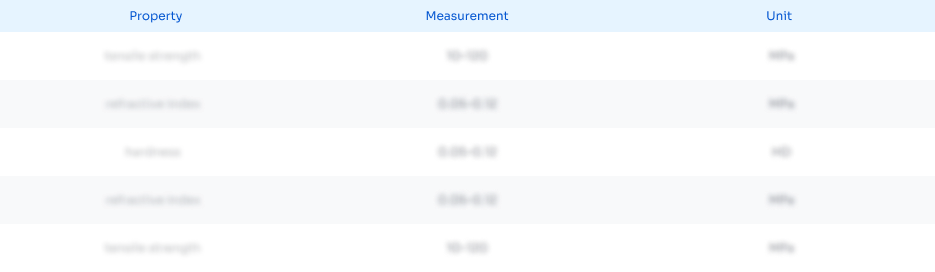
Abstract
Description
Claims
Application Information
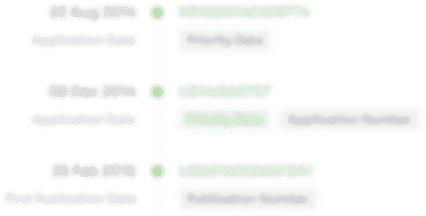
- R&D
- Intellectual Property
- Life Sciences
- Materials
- Tech Scout
- Unparalleled Data Quality
- Higher Quality Content
- 60% Fewer Hallucinations
Browse by: Latest US Patents, China's latest patents, Technical Efficacy Thesaurus, Application Domain, Technology Topic, Popular Technical Reports.
© 2025 PatSnap. All rights reserved.Legal|Privacy policy|Modern Slavery Act Transparency Statement|Sitemap|About US| Contact US: help@patsnap.com