Prediction method for residual bearing capacity and crack propagation path of crack-containing structure based on LSTM-cGAN
A technology of crack propagation and prediction method, applied in the intersection of civil and structural engineering and computer vision, can solve problems such as unsatisfactory simulation effect and incorrect physical model, and achieve the effect of high accuracy and fast calculation speed
- Summary
- Abstract
- Description
- Claims
- Application Information
AI Technical Summary
Problems solved by technology
Method used
Image
Examples
Embodiment Construction
[0034] The purpose and effects of the present invention will become clearer by describing the present invention in detail according to the accompanying drawings and preferred embodiments. It should be understood that the specific embodiments described here are only used to explain the present invention, not to limit the present invention.
[0035] The present invention provides a prediction method based on LSTM-cGAN for the remaining bearing capacity of cracked structures and crack propagation paths, and applies the latest algorithm conditional generation adversarial network in the field of deep learning. The conditional generation adversarial network is proved to be able to efficiently process images and Generate an image. The long-short-term memory method adopted in the present invention is proved to be the most suitable algorithm for dealing with timing problems among the existing machine learning algorithms. The invention applies the latest algorithm in the field of deep ...
PUM
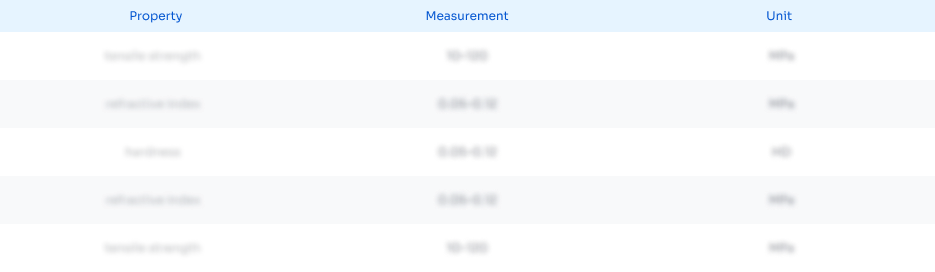
Abstract
Description
Claims
Application Information
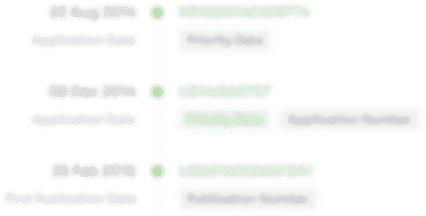
- R&D
- Intellectual Property
- Life Sciences
- Materials
- Tech Scout
- Unparalleled Data Quality
- Higher Quality Content
- 60% Fewer Hallucinations
Browse by: Latest US Patents, China's latest patents, Technical Efficacy Thesaurus, Application Domain, Technology Topic, Popular Technical Reports.
© 2025 PatSnap. All rights reserved.Legal|Privacy policy|Modern Slavery Act Transparency Statement|Sitemap|About US| Contact US: help@patsnap.com