Rolling bearing fault diagnosis method based on short-time Hilbert transform
A rolling bearing and fault diagnosis technology, applied in neural learning methods, testing of mechanical components, testing of machine/structural components, etc., can solve problems such as inaccurate characteristic parameters of fault frequency band positioning, and reduce the influence of artificial extraction of features. Effects of Capture, Strong Adaptive and Robust Features
- Summary
- Abstract
- Description
- Claims
- Application Information
AI Technical Summary
Problems solved by technology
Method used
Image
Examples
Embodiment 1
[0054] Embodiment 1, a rolling bearing fault diagnosis method based on short-time Hilbert transform, such as figure 1 As shown, taking the acceleration data of the drive end collected on the bearing simulation test bench at a rotation speed of 4170r / min as an example, it contains 9 classification results, and the classification results are normal, slight inner ring fault, moderate inner ring fault, Outer ring minor fault, outer ring moderate fault, rolling element minor fault, rolling element moderate fault, cage minor fault and cage moderate fault.
[0055] The method steps include:
[0056] A) Acquisition of bearing vibration signal y(n)=h(n)*x(n)+e(n), n=1,2,...,N, the time domain diagram of the collected bearing vibration signal is as follows figure 2 As shown, where h(n) is the transfer function, x(n) is the shock sequence of the bearing vibration signal, and e(n) is the noise. Using minimum entropy deconvolution to design an optimal filter, the steps include:
[0057...
PUM
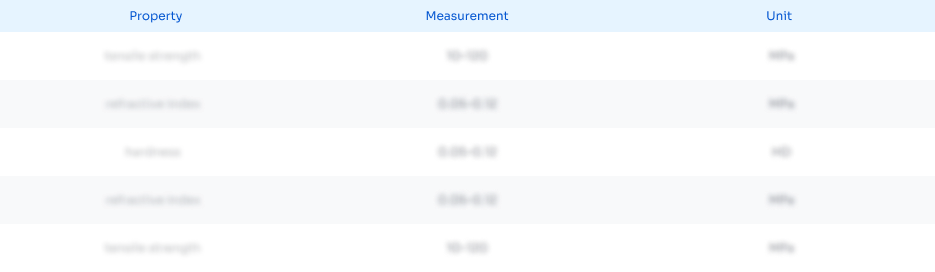
Abstract
Description
Claims
Application Information
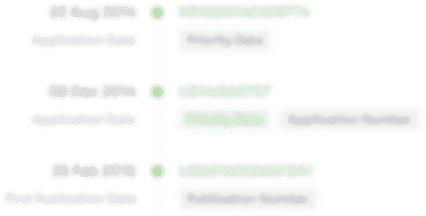
- R&D Engineer
- R&D Manager
- IP Professional
- Industry Leading Data Capabilities
- Powerful AI technology
- Patent DNA Extraction
Browse by: Latest US Patents, China's latest patents, Technical Efficacy Thesaurus, Application Domain, Technology Topic, Popular Technical Reports.
© 2024 PatSnap. All rights reserved.Legal|Privacy policy|Modern Slavery Act Transparency Statement|Sitemap|About US| Contact US: help@patsnap.com