Virtual power plant economic dispatching method in energy internet based on deep reinforcement learning
An energy Internet and reinforcement learning technology, applied in the field of energy distribution of virtual power plants, can solve problems such as high computational complexity, large communication load and delay, and poor reliability of data transmission, so as to preserve privacy, reduce communication load, and reduce computational complexity degree of effect
- Summary
- Abstract
- Description
- Claims
- Application Information
AI Technical Summary
Problems solved by technology
Method used
Image
Examples
specific Embodiment approach 1
[0024] Specific implementation mode 1: The economic scheduling method of virtual power plants in the energy Internet based on deep reinforcement learning described in this implementation mode, the method includes the following steps:
[0025] Step 1. For any area i, use the industrial side server and power supply side server of area i to collect the power generation side and user side information from area i, i=1,2,...,I, I is the total number of areas;
[0026] Use the information collected in each region to train the actor-critic network of the cloud server of the VPP operator, so as to obtain the actor-critic network trained by using the information of each region;
[0027] Step 2. Deploy the trained actor-critic network on the edge nodes of the corresponding regions;
[0028] Step 3: The industrial-side servers and power-side servers in each region collect information from the power generation side and the user side in real time, and input the collected information into th...
specific Embodiment approach 2
[0029] Specific embodiment 2: The difference between this embodiment and specific embodiment 1 is that in the first step, the actor-critic network of the VPP operator cloud server is trained using the information collected in each area, and an asynchronous method is adopted. , running 8 threads in parallel at the same time.
specific Embodiment approach 3
[0030] Embodiment 3: The difference between this embodiment and Embodiment 1 is that the objective function of the actor-critic network is:
[0031]
[0032] Where: C is the total operating cost of area i, is the initial depreciation cost of photovoltaic investment in region i at time slot k, k=0,1,...,K (considering 24 hours in VPP, K is equal to 23), is the photovoltaic operation and maintenance cost of area i in time slot k, is the initial depreciated cost of wind turbines in region i at time slot k, is the wind turbine operation and maintenance cost in region i at time slot k, is the initial depreciation cost of the micro gas turbine in region i at time slot k, is the operation and maintenance cost of the micro gas turbine in region i at time slot k, is the environmental cost of micro gas turbines in region i at time slot k, is the cost consumed by the micro gas turbine in area i at time slot k, λ is the compensation coefficient, is the controllable load ...
PUM
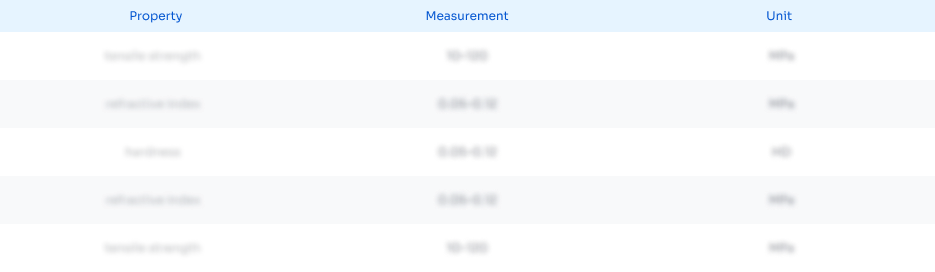
Abstract
Description
Claims
Application Information
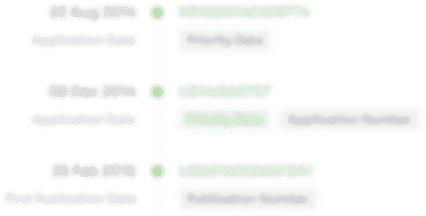
- R&D Engineer
- R&D Manager
- IP Professional
- Industry Leading Data Capabilities
- Powerful AI technology
- Patent DNA Extraction
Browse by: Latest US Patents, China's latest patents, Technical Efficacy Thesaurus, Application Domain, Technology Topic, Popular Technical Reports.
© 2024 PatSnap. All rights reserved.Legal|Privacy policy|Modern Slavery Act Transparency Statement|Sitemap|About US| Contact US: help@patsnap.com