Machine learning blood pressure monitoring method based on LSTM neural network
A neural network and machine learning technology, applied in the medical field, can solve the problems of measurement accuracy, low accuracy, pulse wave interference, etc., and achieve the effect of improving universality, high comfort, and convenient detection.
- Summary
- Abstract
- Description
- Claims
- Application Information
AI Technical Summary
Problems solved by technology
Method used
Image
Examples
Embodiment Construction
[0043] The present invention will be described in further detail below in combination with specific embodiments and with reference to the accompanying drawings.
[0044] as attached figure 1 As shown, the present invention first obtains the photoplethysmography, electrocardiogram and pressure pulse wave signals.
[0045] The acquisition of the above signals mainly includes the following units: a photoelectric sensor unit, an electrocardiogram unit and a pressure pulse wave unit.
[0046] The photoelectric signal can be obtained by a photoelectric sensor. The principle is to use light-emitting diodes to irradiate human blood vessels. The light is green light with a wavelength of 530nm. Compared with red light and infrared light, green light has stronger penetrating power. The reflection interference is smaller, and the obtained photoplethysmogram waveform is better. Photoelectric sensors can be placed on wrists, fingers, etc. When the green light is reflected to the photodio...
PUM
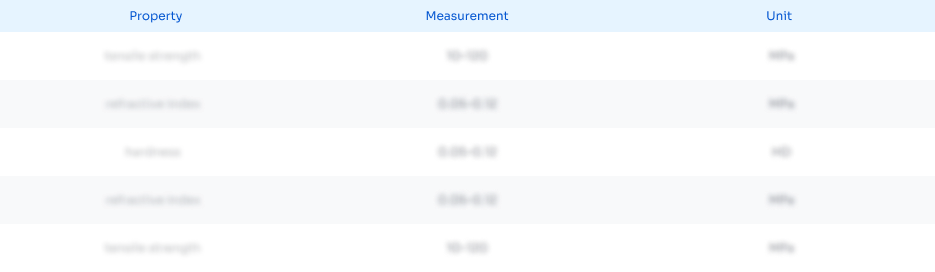
Abstract
Description
Claims
Application Information
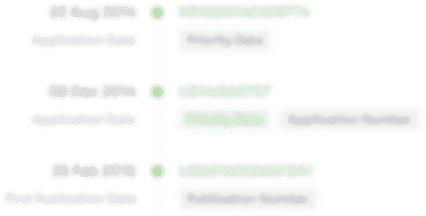
- Generate Ideas
- Intellectual Property
- Life Sciences
- Materials
- Tech Scout
- Unparalleled Data Quality
- Higher Quality Content
- 60% Fewer Hallucinations
Browse by: Latest US Patents, China's latest patents, Technical Efficacy Thesaurus, Application Domain, Technology Topic, Popular Technical Reports.
© 2025 PatSnap. All rights reserved.Legal|Privacy policy|Modern Slavery Act Transparency Statement|Sitemap|About US| Contact US: help@patsnap.com