Medicine relocation method based on deep learning multi-source heterogeneous network
A multi-source heterogeneous and deep learning technology, which is applied in the field of computer bioinformatics network embedding and machine learning, can solve the problem of drug information data sample imbalance, achieve effective prediction and improve the effect of prediction accuracy
- Summary
- Abstract
- Description
- Claims
- Application Information
AI Technical Summary
Problems solved by technology
Method used
Image
Examples
Embodiment Construction
[0026] Below in conjunction with accompanying drawing and specific implementation method, the present invention will be described in further detail:
[0027] A drug repositioning method based on deep learning multi-source heterogeneous network, comprising the following steps:
[0028] Step 1: Calculate the topology information of the multi-source heterogeneous network based on the random walk method, including the following steps:
[0029] 1.1. Input multi-source heterogeneous dataset D=[D 1 ,D 2 ,...,D 9 ], where D represents the multi-source heterogeneous datasets related to drugs, D1, D2, D3,..., D9 respectively represent the drug-drug interaction matrix, drug-target interaction matrix, drug-side effect correlation matrix and 6 kinds of Drug similarity matrix;
[0030] 1.2. Based on the random walk method, calculate and capture the network structure information of the data set in D and describe the topological context of each drug, and obtain the probability co-occurren...
PUM
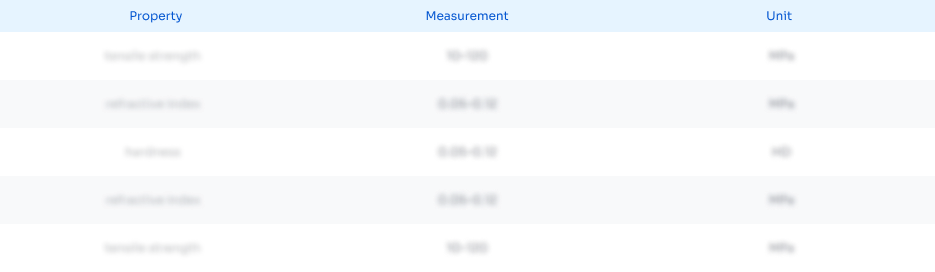
Abstract
Description
Claims
Application Information
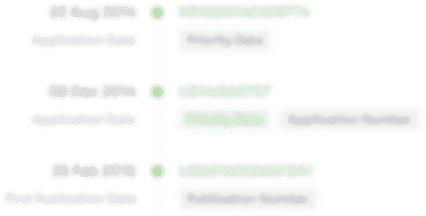
- R&D Engineer
- R&D Manager
- IP Professional
- Industry Leading Data Capabilities
- Powerful AI technology
- Patent DNA Extraction
Browse by: Latest US Patents, China's latest patents, Technical Efficacy Thesaurus, Application Domain, Technology Topic, Popular Technical Reports.
© 2024 PatSnap. All rights reserved.Legal|Privacy policy|Modern Slavery Act Transparency Statement|Sitemap|About US| Contact US: help@patsnap.com